Abderahman Rejeb a , Alireza Abdollahi b , Karim Rejeb c , Horst Treiblmaier d,
- a Department of Management and Law, Faculty of Economics, University of Rome Tor Vergata, Via Columbia, 2, Rome 00133, Italy
- b Department of Business Administration, Faculty of Management, Kharazmi University, 1599964511 Tehran, Iran
- c Faculty of Sciences of Bizerte, University of Carthage, Zarzouna, 7021 Bizerte, Tunisia
- d School of International Management, Modul University Vienna, Am Kahlenberg 1, 1190 Vienna, Austria
ARTICLE INFO | ABSTRACT |
Keywords: Drones UAV Precision agriculture Internet of Things Bibliometrics | Drones, also called Unmanned Aerial Vehicles (UAV), have witnessed a remarkable development in recent decades. In agriculture, they have changed farming practices by offering farmers substantial cost savings, increased operational efficiency, and better profitability. Over the past decades, the topic of agricultural drones has attracted remarkable academic attention. We therefore conduct a comprehensive review based on bibliometrics to summarize and structure existing academic literature and reveal current research trends and hotspots. We apply bibliometric techniques and analyze the literature surrounding agricultural drones to summarize and assess previous research. Our analysis indicates that remote sensing, precision agriculture, deep learning, machine learning, and the Internet of Things are critical topics related to agricultural drones. The co-citation analysis reveals six broad research clusters in the literature. This study is one of the first attempts to summarize drone research in agriculture and suggest future research directions. |
Introduction
Agriculture represents the primary food source of the world (Friha et al., 2021), and it has been facing severe challenges due to the
increasing demand for food products, food safety, and security concerns as well as calls for environmental protection, water preservation, and
sustainability (Inoue, 2020). This development is predicted to continue since the world population is estimated to reach 9.7 billion by 2050
(2019). Since agriculture constitutes the most prominent example of water consumption globally, it is expected that food demand and water
consumption will dramatically increase in the foreseeable future. Furthermore, the increasing consumption of fertilizers and pesticides
coupled with the intensification of farming activities could lead to future environmental challenges. Similarly, arable land is limited, and the
number of farmers is decreasing worldwide. These challenges accentuate the need for innovative and sustainable farming solutions (Elijah
et al., 2018; Friha et al., 2021; Inoue, 2020; Tzounis et al., 2017).
Incorporating novel technologies has been identified as a promising solution to address these challenges. Smart farming (Brewster et al.,
2017; Tang et al., 2021) and precision agriculture (Feng et al., 2019; Khanna & Kaur, 2019) have emerged as a result of such debates. The
former is a general notion for adopting information communication technologies (ICT) and other cutting-edge innovations in farming activities to increase efficiency and efficacy (Haque et al., 2021). The latter focuses on site-specific management in which the land is divided into
homogeneous parts, and each part gets the exact amount of agricultural input for crop yield optimization by means of novel technologies (Feng et al., 2019; Khanna & Kaur, 2019). Prominent technologies that have attracted scholars’ attention in this field include Wireless Sensor Networks (WSNs) (J. Zheng & Yang, 2018; Y. Zhou et al., 2016), the Internet of Things (IoT) (Gill et al., 2017; He et al., 2021; Liu et al., 2019),
artificial intelligence (AI) techniques, including machine learning and deep learning (Liakos et al., 2018; Parsaeian et al., 2020; Shadrin et al.,
2019), computing technologies (Hsu et al., 2020; Jinbo et al., 2019; Zamora-Izquierdo et al., 2019), big data (Gill et al., 2017; Tantalaki
et al., 2019), and blockchain (P. W. Khan et al., 2020; Pincheira et al., 2021).
In addition to the abovementioned technologies, remote sensing has been considered a technological tool with high potential to improve
smart and precision agriculture. Satellites, human-crewed aircraft, and drones are popular remote-sensing technologies (Tsouros et al., 2019).
Drones, popularly known as Unmanned Aerial Vehicles (UAVs), Unmanned Aircraft Systems (UAS), and remotely piloted aircraft, are of
great importance as they have multiple advantages in comparison with other remote-sensing technologies. For example, drones can deliver
high-quality and high-resolution images on cloudy days (Manfreda et al., 2018). Also, their availability and transfer speed constitute other
benefits (Radoglou-Grammatikis et al., 2020). Compared with aircraft, drones are highly cost-efficient and easy to set up and maintain (Tsouros et al., 2019). Despite being initially mainly used for military purposes, drones can benefit numerous civilian applications, for example in supply chain management (A. Rejeb, Rejeb, et al., 2021a), for humanitarian purposes (A. Rejeb, Rejeb, et al., 2021c), smart agriculture, surveying and mapping, cultural heritage documentation, disaster management, and forest and wildlife conservation (Panday, Pratihast, et al., 2020). In agriculture, manifold application areas of drones exist as they can be integrated with novel technologies, computing capabilities, and onboard sensors to support crop management (e.g., mapping, monitoring, irrigation, plant diagnosis) (H. Huang et al., 2021), disaster reduction, early warning systems, wildlife and forestry conservation to name a few (Negash et al., 2019). Similarly, drones could be leveraged in several agricultural activities, including crop and growth monitoring, yield estimation, water stress assessment, and weeds, pest, and disease detection (Inoue, 2020; Panday, Pratihast, et al., 2020). Not only can drones be used for monitoring, estimation, and detection purposes based on their sensory data, but also for precision irrigation and precision weed, pest, and disease management. In other words, drones are able to spray water and pesticides in precise amounts based on environmental data. The benefits of drones in agriculture are summarized in Table 1.
Main benefits of drones in agriculture.
Benefit | Reference(s) |
Enhance temporal and spatial sensing resolutions | (Gago et al., 2015; Niu et al., 2020; Srivastava et al., 2020) |
Facilitate precision agriculture | (L. Deng et al., 2018; Kalischuk et al., 2019; Maimaitijiang et al., 2017) |
Classification and scouting of crops | (Inoue, 2020; Kalischuk et al., 2019; Lopez- ´ Granados et al., 2016; Maimaitijiang et al., 2017; Melville et al., 2019; Moharana & Dutta, 2016) |
Usage of fertilizer | (L. Deng et al., 2018; Guan et al., 2019) |
Monitoring of drought | (Fawcett et al., 2020; Panday, Pratihast, et al., 2020; Su et al., 2018) |
Biomass estimation | (Bendig et al., 2014) |
Yield estimation | (Inoue, 2020; Panday, Shrestha, et al., 2020; Tao et al., 2020) |
Disaster reduction | (Negash et al., 2019) |
Conservation of wildlife and forestry | (Negash et al., 2019; Panday, Pratihast, et al., 2020) |
Assessment of water stress | (Inoue, 2020; J. Su, Coombes, et al., 2018; L. Zhang et al., 2019) |
Pest, weeds, and disease detection | (Gaˇsparovi´c et al., 2020; Inoue, 2020; J. Su, Liu, et al., 2018; X. Zhang et al., 2019) |
On the other hand, drones face limitations as well. Pilot involvement, engine power, stability and reliability, sensors’ quality due to payload
weight limitations, implementation costs, and aviation regulation, are among them (C. Zhang & Kovacs, 2012). We compare the shortcomings
of the three mobile remote sensing technologies in Table 2. Other remote sensing technologies, such as soil sensors, are beyond the focus of this study.
Shortcomings of various mobile remote sensing technologies.
Remote sensing technologies | Shortcomings | References |
Drone (UAV) | Pilot involvement; images’ quality (average); implementation costs (average); stability, maneuverability, and reliability; standardization; engine power; limited power sources (battery longevity); limited flight duration, collision and cyberattacks; limited payload weight; large datasets and limited data processing capabilities; lack of regulation; lack of expertise, high entry barriers to the access to agricultural drones; | (Bacco et al., 2018; Dawaliby et al., 2020; Hardin & Hardin, 2010; Hardin & Jensen, 2011; Lagkas et al., 2018; Laliberte et al., 2007; Laliberte & Rango, 2011; Manfreda et al., 2018, 2018; Nebiker et al., 2008; Puri et al., 2017; Velusamy et al., 2022; C. Zhang & Kovacs, 2012) |
Satellite | Periodic satellite coverage, limited spectral resolution; vulnerability to visibility issues (e.g., clouds); Unavailability and low transfer speed; orientation and vignetting effects costly spatial data collection; slow data delivery time to end users | (Aboutalebi et al., 2019; Cen et al., 2019; Chen et al., 2019; Nansen & Elliott, 2016; Panday, Pratihast, et al., 2020; Sai Vineeth et al., 2019) |
Aircraft | High adoption costs; complicated set-up; maintenance costs; unavailability of reliable airplanes, geometry of the images; non-regular data acquisition; lack of flexibility; deadly accidents; sensor data variations due to vibrations; georeferencing issues | (Armstrong et al., 2011; Atkinson et al., 2018; Barbedo & Koenigkan, 2018; Kovalev & Voroshilova, 2020; Suomalainen et al., 2013; Thamm et al., 2013) |
As a multidisciplinary and multipurpose technology in agriculture, drones have been investigated from various perspectives. For example, scholars have examined drone applications in agriculture (Kulbacki et al., 2018; Mogili & Deepak, 2018), their contribution to precision agriculture (Puri et al., 2017; Tsouros et al., 2019), their complementarity with other cutting-edge technologies (Al-Thani et al., 2020; Dutta & Mitra, 2021; Nayyar et al., 2020; Saha et al., 2018), and the possibilities of advancing their navigational and sensing capabilities (Bareth et al., 2015; Suomalainen et al., 2014). Since research on drone applications in agriculture has become prevalent (Khan et al., 2021)), there is a need to summarize the extant literature and reveal the domain’s intellectual structure. Furthermore, as a high-tech field with continuous improvements, structured reviews need to be conducted to periodically summarize the extant literature and identify important research gaps. To
date, there are few reviews that discuss drone applications in the agricultural sector. For example, Mogili and Deepak (2018) briefly reviewdrones’ implications for crop monitoring and pesticide spraying. Inoue (2020) conducts a review of satellite and drone use in remote sensing in agriculture. The author explores the technological challenges of adopting smart farming and the contributions of satellites and drones based on case studies and best practices. Tsouros et al. (2019) summarize different types of drones and their main applications in agriculture, highlighting various data acquisition and processing methods. More recently, Aslan et al. (2022) conducted a comprehensive review of UAV applications in agricultural activities and underscored the relevance of simultaneous localization and mapping for a UAV in the greenhouse. Diaz-Gonzalez et al. (2022) reviewed recent studies of crop yield production based on different machine learning techniques and remote
sensing systems. Their findings indicated that UAVs are useful to estimate soil indicators and outperform satellite systems in terms of spatial resolution, information temporality, and flexibility. Basiri et al. (2022) made an exhaustive review of the various approaches and methods to overcome path-planning challenges for multi-rotor UAVs in the context of precision agriculture. Moreover, Awais et al. (2022) summarized the application of UAV remote sensing data in crops to estimate the water status and provided an in-depth synthesis of the prospective capacity of UAV remote sensing for waster stress application. Finally, Aquilani et al. (2022) reviewed prevision farming technologies applied in pasturebased livestock systems and deduced that remote sensing enabled by UAVs is advantageous for biomass assessment and herd management.
Also, endeavors to use UAVs in the monitoring, tracking, and mustering of livestock have been reported recently.
Although these reviews yield new and important insights, no comprehensive and up-to-date review based on bibliometrics can be found in the literature, which presents a clear knowledge gap. Moreover, it has been stated that when scholarly production grows in a scientific domain, it becomes vital for researchers to employ quantitative review approaches to comprehend the knowledge structure of the domain (Rivera & Pizam, 2015). Similarly, Ferreira et al. (2014) argued that as research fields mature and become intricate, scholars should aim to occasionally make sense of the knowledge generated and amassed to reveal new contributions, capture research traditions and trends, identify which topics are studied, and delve into the knowledge structure of the field and the potential research directions. While Raparelli and Bajocco (2019) conducted a bibliometric analysis to examine the knowledge domain of drone applications in agriculture and forestry, their study only considers scholarly research published between 1995 and 2017, which does not reflect the dynamics of this fast-moving area. Further, the authors did not attempt to identify the most influential contributions in the field, cluster the literature, and evaluate the intellectual structure using co-citation analysis. As a result, it is necessary to summarize the literature to reveal current research foci, trends, and hotspots.
To fill this knowledge gap, we leverage quantitative methodology and rigorous bibliometric methods to examine the current state of research at the intersection of drones and agriculture. We argue that the current study makes several contributions to the existing literature by examining an emerging technology which is highly needed in agriculture as it provides tremendous potential to alter several aspects in this sector. The need for a bibliometric analysis of agricultural drones is sensed even more given the scattered and fragmented knowledge on drones within the agriculture context. Similarly, the literature pertaining to agricultural drones is required to be systematically clustered, considering the most influential studies which build the foundation of this research field. The merit in the analysis also includes the clarification of main research themes represented in the literature. Considering the transformational potential of the technology, we posit that an indepth network analysis yields novel insights by determining influential works and revealing themes concerning drones’ potential for agriculture.
We therefore strive to achieve the following research objectives:
- Identification of influential publications with outstanding contributions to drone applications in the field of agriculture.
- Clustering of the literature, identification of research foci, and mapping of the main ‘intellectual structure’ studies based on semantic similarity by using co-citation analysis.
- Understanding of the evolution of linkages and citation networks over time among various publications in the field and identification of future research directions and hot topics.
The rest of the paper is structured as follows: section 2 outlines the methodology and data collection steps; section 3 provides the results of the analyses; and section 4 discusses the findings and concludes with research contributions, implications, and future directions.
Methodology
In this current research study, we conduct a bibliometric analysis to explore drone applications in agriculture. This quantitative approach reveals the intellectual structure of the knowledge domain (Arora & Chakraborty, 2021) and the current status, hot topics, and future research directions that can be investigated by applying this method (Kapoor et al., 2018; Mishra et al., 2017; A. Rejeb, Rejeb, et al., 2021b; A. Rejeb et al., 2021d; M. A. Rejeb et al., 2020). Generally, a bibliometric analysis examines the extant literature to summarize and uncover hidden patterns of written communication and the evolution of the discipline based on statistics and mathematical methods, and it applies to large data sets (Pritchard, 1969; Small, 1999; Tahai & Rigsby, 1998). By using bibliometrics, we aspire to better understand the existing paradigms and research foci that contribute to the domain based on similarity (Thelwall, 2008). Bibliometrics provides new insights backed by the objective quantitative strength of the methodology (Casillas & Acedo, 2007). Numerous scholars have previously conducted bibliometric studies in related domains, including agriculture, remote sensing, and digital transformation (Armenta-Medina et al., 2020; Bouzembrak et al., 2019; A. Rejeb, Treiblmaier, et al., 2021; Wamba & Queiroz, 2021; Wang et al., 2019).
Citation analysis
Citation analysis reveals various insights into a given research field. First of all, it helps to reveal the most influential authors and publications that contribute to a given research field and make a significant impact (Gundolf & Filser, 2013). Secondly, the knowledge flow and the communication links between authors can be uncovered. Finally, by tracing the links between cited and citing works, one can explore the changes and evolution of a knowledge domain over time (Pournader
et al., 2020). High citation numbers of a publication reflect its relevance and substantial contributions to the research domain (Baldi, 1998; Gundolf & Filser, 2013; Marinko, 1998). Citation analysis of publications also helps to identify relevant works and track their popularity and progress over time.
Document co-citation analysis
Co-citation analysis is a valuable method to explore relationships between publications and depict the intellectual structure of a field (Nerur et al., 2008). In other words, by identifying the most cited publications and their connections, the method groups publications into distinct research clusters wherein publications in a cluster regularly share similar ideas (McCain, 1990; Small, 1973). It is crucial to mention that similarity does not mean that the findings of the publications are
cohesive and agree with each other; publications belong to the same cluster due to topic similarity, but they can have contradicting viewpoints.
Data collection and analysis
Following the methodology proposed by White and Griffith (1981), we performed a comprehensive search of journal articles to cover the entire research domain of drone applications in agriculture, pursuing the following five steps:
- The first step was data collection. Scopus was selected as one of the most comprehensive and trustworthy databases with standardized results. The meta-data of publications related to all drone applications in agriculture was retrieved. Then we analyzed the selected articles, removing off-topic articles from the analysis.
- We analyzed the literature and identified the most important keywords used in the research area.
- Using citation analysis, we explored the connection between authors and documents to reveal underlying citation patterns. We also identified the most influential authors and publications with significant contributions to the field of agricultural drones.
- We conducted a co-citation analysis to group similar publications into clusters.
- Finally, we analyzed the connections and linkages between countries, institutions, and journals to depict the collaboration network.
Identification of appropriate search terms
We applied the following search strings for data aggregation: (drone* OR “unmanned aerial vehicle” OR uav* OR “unmanned aircraf system” OR uas OR “remotely piloted aircraft”) AND (agricultural OR agriculture OR farming OR farmer). The search was conducted in September 2021. Drones have several designations, including UAV, UAS, and remotely piloted aircraft (Sah et al., 2021). The specific search terms related to agriculture were identified based on the study of Abdollahi et al. (2021). For the sake of clarity and transparency, the exact query we used is given in Appendix 1. Following a data cleaning process, we created a text file that was subsequently loaded into BibExcel, a common tool for citation and co-citation analysis. This tool also offers simple interaction with other software and offers a significant degree of freedom in data handling and analysis. VOSviewer version 1.6.16 was used to visualize the findings and generate the bibliometric networks (Eck & Waltman, 2009). VOSviewer offers a range of intuitive visualization, particularly for analyzing bibliometric maps (Geng et al., 2020). Furthermore, it assists in providing plain visual results that aid in better understanding the results (Abdollahi et al., 2021). Applying the search strings as stated above, we gathered and stored all relevant publications. The first search results yielded a total of 5,085 documents. To ensure the quality of the selected sample, only peer-reviewed journal articles were considered in the research, resulting in the exclusion of other document types, such as books, chapters, conference proceedings, and editorial notes. During a screening process, irrelevant (i.e., beyond the scope of this work), redundant (i.e., duplicates originating from double indexing), and non-English-speaking publications were filtered out. This process resulted in the inclusion of 4,700 documents in the final analysis.
Findings and discussion
To start, we analyzed the developments in publication output in the current literature on agricultural drones. The temporal distribution of scholarly research is shown in Fig. 1. We see a rapid increase in publications from the year 2011 (30 publications) onward; therefore, we decided to split the analysis period into two different stages. We refer to the period between 1990 and 2010 as the build-up stage, which had roughly seven papers published annually. The post-2010 period has been called the growth stage since research on drone applications in agriculture witnessed an exponential surge during this period. After 2010, the increasing number of publications confirms the growing interest among researchers, which also reflects that drones have been applied to remote sensing and used in precision agriculture (Deng et al., 2018; Maes & Steppe, 2019; Messina & Modica, 2020). Specifically, the number of publications rose from 108 in 2013 to 498 in 2018 and peaked at 1,275 in 2020. A total of 935 articles were published between January and mid-September 2021. Subsequently, we opted to focus our analysis more on the growth stage since this period reflects the most recent and important subtleties of agricultural drones.
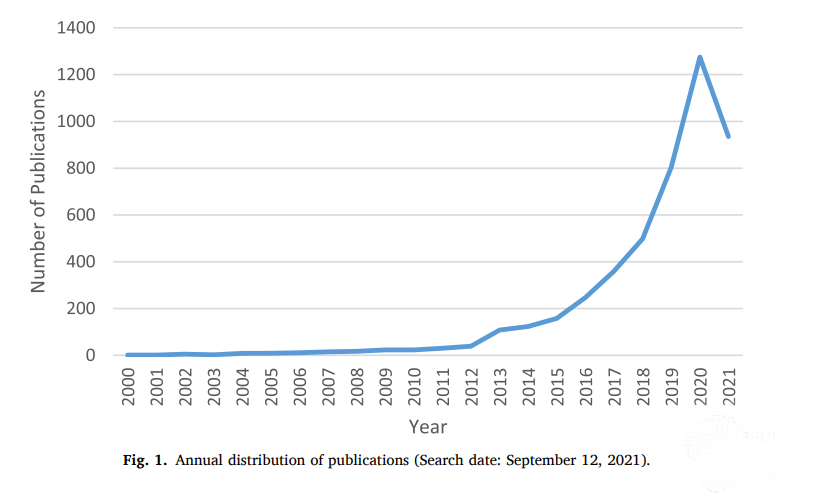
Keywords analysis
The keywords authors select for a publication have a crucial impact on how the paper is represented and how it is communicated within scientific communities. They identify the key subjects of the research and determine its potential to flourish or fail (Day & Gastel, 1998.; Kim et al., 2016; Uddin et al., 2015). Keywords analysis, a tool to reveal broader research trends and directions, refers to the compilation of the keywords of all related publications in a domain (Dixit & Jakhar, 2021). In the current study, we divided the aggregated keywords into two sets (i.e., until 2010 and 2011–2021) to explore the most popular topics. By doing this, we can trace the crucial keywords in both sets and assure that we captured all the necessary data. For each set, the top ten keywords are presented in Table 3. We eliminated inconsistencies by merging semantically identical keywords, such as “drone” and “drones” or, similarly, “Internet of Things” and “IoT.”.
Table 3 shows that “unmanned aerial vehicle” is a more frequently used keyword as compared to “drone” and “unmanned aerial system” in both time periods. Also, “remote sensing,” “precision agriculture,” and “agriculture” are highly ranked in both periods. In the first period, “precision agriculture” ranked fifth, and it ranked second in the second period, which illustrates how drones are becoming increasingly important in achieving precision agriculture as they can make monitoring,
detection, and estimation practices faster, cheaper, and easier to perform in comparison with other remote-sensing and ground-based systems. Also, they can spray the precise amount of input (e.g., water or pesticides) when needed (Guo et al., 2020; Inoue, 2020; Panday, Pratihast, et al., 2020).
List of most frequently used keywords.
Rank | 1990–2010 | No. of occurrences | 2011–2021 | No. of occurrences |
1 | unmanned aerial vehicle | 28 | unmanned aerial vehicle | 1628 |
2 | remote sensing | 7 | precision agriculture | 489 |
3 | agriculture | 4 | remote sensing | 399 |
4 | airborne | 4 | drone | 374 |
5 | precision agriculture | 4 | unmanned aerial system | 271 |
6 | unmanned aerial | 4 | agriculture | 177 |
7 | hyperspectral sensor | 3 | deep learning | 151 |
8 | artificial neural networks | 2 | machine learning | 149 |
9 | autonomous fligh | 2 | vegetation Index | 142 |
10 | coffee | 2 | Internet of Things | 124 |
Another interesting feature is the presence of complementary technologies. In the first stage, “Hyperspectral Sensor” and “artificial neural networks” (ANN) are among the top ten keywords. Hyperspectral imaging revolutionized traditional imaging by collecting a huge number of images at various wavelengths. In doing so, the sensors can simultaneously collect better spatial and spectral information as compared to multispectral imaging, spectroscopy, and RGB imagery (Adao ˜ et al.,
2017). The occurrence of “ANN” in the first stage and “deep learning” (DL) and “machine learning” (ML) in the second one implies that most of the published works focused on the examination of the potential of AI techniques for drone-based agriculture. Although drones are capable of flying autonomously, they still require the involvement of a pilot, which implies a low level of device intelligence. However, this problem can be solved due to the advancement of AI techniques, which can provide better situational awareness and autonomous decision support. Equipped with AI, drones can avoid collisions during navigation, improve soil and crop management (Inoue, 2020), and reduce labor and stress for human beings (B. K. Sharma et al., 2019).
Due to their flexibility and ability to handle vast amounts of nonlinear data, AI techniques are suitable methods to analyze the data transmitted by drones and other remote-sensing and ground-based systems for prediction and decision-making (Ali et al., 2015; Inoue, 2020). Furthermore, the presence of “IoT” in the second period indicates its emerging role in agriculture. IoT is revolutionizing agriculture by interconnecting other technologies, including drones, ML, DL, WSNs, and big data. One of the key benefits of implementing IoT is its ability to efficiently and effectively merge various tasks (data acquisition, data analysis and processing, decision-making, and implementation) in near real time (Elijah et al., 2018; Feng et al., 2019; Muangprathub et al., 2019). Furthermore, drones are considered efficient tools for capturing the data necessary for calculating vegetation’s vigor and vegetation properties (Candiago et al., 2015). Fig. 2a and 2b illustrate the keyword co-occurrence networks for both time periods.
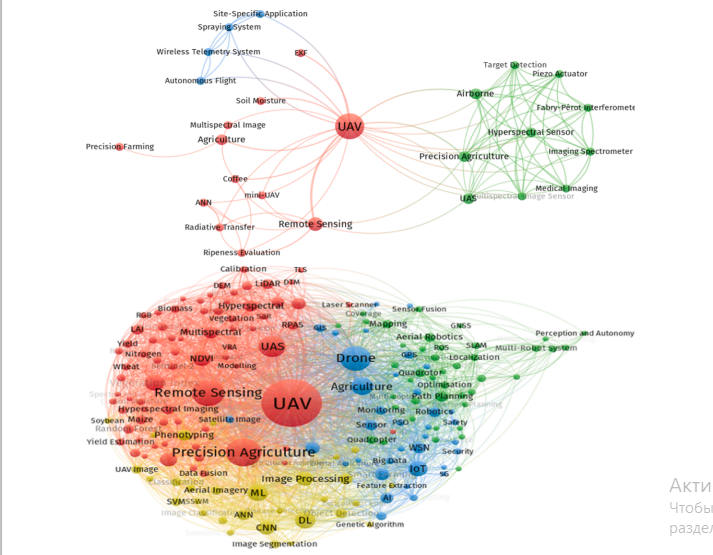
Influential authors
In this section, we determine the influential authors and examine how author citation networks can visualize and organize the current literature. Fig. 3 shows the chronological overlay of all researchers with the highest number of citations. The color scale reflects the year-wise variation of authors’ citations. We examine the citation structure of researchers who published studies on agricultural drones by using a threshold of a minimum of 50 citations and ten publications. Out of
12,891 authors, only 115 met this condition. Table 4 lists the top ten influential authors, sorted by the maximum number of citations. Lopez- Granados F. leads the list with 1,963 citations, followed by Zarco-Tejada P.J. with 1,909 citations.
List of most cited authors.
Ranking | Author | Citations |
1 | Lopez-Granados ´ F. | 1,963 |
2 | Zarco-Tejada P.J. | 1,909 |
3 | Pena ˜ J.M. | 1,644 |
4 | Torres-S´ anchez J. | 1,576 |
5 | Fereres E | 1,339 |
6 | Remondino F | 1,235 |
7 | Bolten A | 1,160 |
8 | Bareth G | 1,155 |
9 | Berni J.A | 1,132 |
10 | de Castro A.I. | 1,036 |
When it comes to individual publications, Zhang and Kovacs’s (2012) article has been the most cited study published in Precision Agriculture. Herein, the authors reviewed the application of UAS in precision agriculture. The findings of their research suggest that there is a need to advance platform design, production, standardization of image georeferencing, and information retrieval workflow to provide farmers with reliable end products. Additionally, they recommend engaging the farmer more strongly, especially in field planning, image capture, as well as data interpretation and analysis. Importantly, this study was among the first to show the importance of UAV in field mapping, vigor mapping, chemical content measurement, vegetation stress monitoring, and evaluation of effects of fertilizers on plant growth. The challenges related to the technology also include prohibitive costs, sensor capability, platform stability and reliability, lack of standardization, and consistent procedure to analyze massive amounts of data.
Citation analysis
Citation analysis represents the study of the influence of articles, albeit prone to flows (e.g., citation bias, self-citation) is considered one of the standard instruments for the impact evaluation (Osareh, 1996; A. Rejeb et al., 2022; Sarli et al., 2010). Citations also reflect the importance and vitality of the papers’ contributions to the literature on a specific topic (R. Sharma et al., 2022). We conducted a citation analysis to determine the most influential studies on agricultural drones and summarized the contents. Table 5 presents the list of the fifteen most influential papers for the periods 1990–2010 and 2011–2021. The articles by Berni et al. (2009)b and Austin (2010) have been the most cited during 1990 and 2010, with 831 and 498 citations, respectively. Berni et al. (2009)b illustrated the potential to develop quantitative remotesensing products via a helicopter-based UAV outfitted with affordable thermal and narrowband multispectral imaging sensors. Compared to traditional manned airborne sensors, a low-cost UAV system for agriculture is able to achieve comparable estimations of the biophysical parameters of crops, if not better. The affordable cost and operational flexibility, alongside the high spectral, spatial, and temporal resolutions available at a quick turnaround time, render UAVs suitable for a range of applications that require time-critical management, including irrigation scheduling, and precision farming. The paper from Berni et al. (2009)b is highly cited because it effectively integrated an unmanned rotary-wing platform and digital and thermal sensors with the necessary calibration mechanisms for agricultural applications. The second most cited publication is a book authored by Austin (2010), who discussed UAVs from design, development, and deployment perspectives. In agriculture, UAVs support crop monitoring by detecting diseases early through crop color changes, facilitating crop sowing and spraying, and monitoring and driving herds.
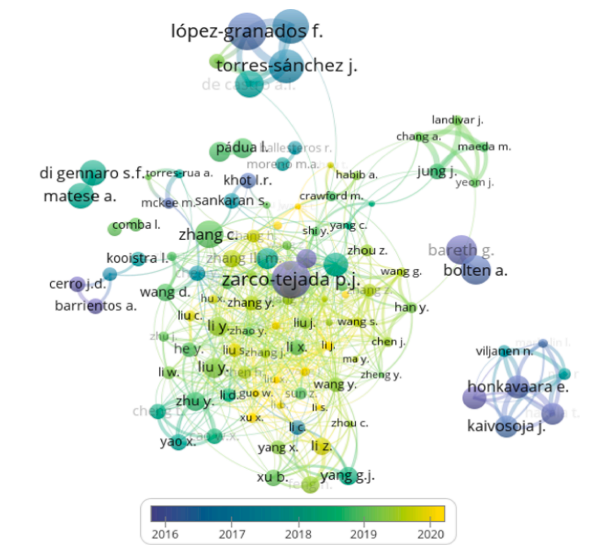
The studies of Sullivan et al. (2007), Lumme et al. (2008), and Gokto ¨ ǧan et al. (2010) finish the list of the top fifteen most cited articles. These articles illustrate the development of UAV-based systems to support agriculture. They offer solutions to various problems, such as crop monitoring and scanning, weed surveillance and management, and decision support. They also suggest and discuss UAV’s ability to increase sampling efficiency and aid farmers in devising accurate and effective
planting strategies. Two papers were authored by Berni (Berni et al., 2009b; Berni et al., 2009a), underscoring his significant impact on agricultural drone-related research. The paper from Zarco-Tejada et al. (2014) has been among the pioneering studies to illustrate the need to use low-cost UAV imagery in tree height quantification.
List of most cited publications.
Rank | From 1990 to 2010 | From 2011 to 2021 | ||
Document | Citation | Document | Citation | |
1 | (Berni et al., 2009b) | 831 | (C. Zhang & Kovacs, 2012) | 967 |
2 | (Austin, 2010) | 498 | (Nex & Remondino, 2014) | 893 |
3 | (Hunt et al., 2010) | 331 | (Floreano & Wood, 2015) | 552 |
4 | (S. R. Herwitz et al., 2004) | 285 | (Hossein Motlagh et al., 2016) | 391 |
5 | (C. C. D. Lelong et al., 2008) | 272 | (Shakhatreh et al., 2019) | 383 |
6 | (Berni et al., 2009b) | 250 | (Ma et al., 2017) | 373 |
7 | (Grenzdorffer ¨ et al., 2008) | 198 | (Bendig et al., 2014) | 360 |
8 | (Hrabar et al., 2005) | 175 | (Zarco-Tejada et al., 2014) | 347 |
9 | (Y. Huang et al., 2009) | 129 | (Ad˜ ao et al., 2017) | 335 |
10 | (Schmale III et al., 2008) | 119 | (Honkavaara et al., 2013a) | 331 |
11 | (Abd-Elrahman et al., 2005) | 79 | (Candiago et al., 2015) | 327 |
12 | (Techy et al., 2010) | 69 | (Xiang & Tian, 2011) | 307 |
13 | (Sullivan et al., 2007) | 51 | (Matese et al., 2015) | 303 |
14 | (Lumme et al., 2008) | 42 | (Gago et al., 2015) | 275 |
15 | (Gokto ¨ ǧan et al., 2010) | 40 | (Aasen et al., 2015a) | 269 |
In the second period (2011–2021), the research by Zhang and Kovacs (2012) and Nex and Remondino (2014) resulted in the most frequently cited publications. Zhang and Kovacs (2012) argue that precision agriculture could benefit from implementing geospatial techniques and sensors, such as geographic information systems, GPS, and remote sensing, to capture variations in the field and handle them by employing alternative strategies. As a game-changer in precision agriculture, the adoption of drones has heralded a new age in remote sensing, simplifying aerial observation, capturing crop growth data, soil conditions, and spraying areas. The review of Zhang and Kovacs (2012) is seminal since it offers insights into UAVs by revealing existing uses and challenges of these devices in environmental monitoring and precision agriculture, such as platform and camera limitations, data processing challenges, farmer engagement, and aviation regulations. The second
most cited study from Nex and Remondino (2014) reviewed the state of the art of UAVs for capturing, processing, and analyzing earth images.
Their work also presented an overview of several UAV platforms, applications, and use cases, showcasing the newest advancements in UAV image processing. In agriculture, farmers could use UAVs to make effective decisions to achieve cost and time savings, receive a rapid and precise record of damages, and anticipate possible problems. In contrast to conventional aerial platforms, UAVs can cut operational expenses and lessen the danger of access in harsh locations while still preserving high precision potential. Their paper summarizes various advantages of UAVs, particularly in terms of accuracy and resolution.
Among the remaining thirteen most cited publications between 2011 and 2021, we noticed a greater concentration on research connected to drone applications in imaging missions (Bendig et al., 2014; Ma et al., 2017; Zarco-Tejada et al., 2014), precision agriculture (Candiago et al., 2015; Honkavaara et al., 2013a), precision viticulture (Matese et al., 2015), water stress assessment (Gago et al., 2015), and vegetation monitoring (Aasen et al., 2015a). In the early years, researchers focused
more on developing low-cost, lightweight, and precise UAV-based systems for agriculture; more recent research has focused more on reviews of UAV applications for agriculture and field surveying. In summary, this analysis reveals that the influential publications have mostly provided reviews of prior studies to evaluate UAVs’ current scientific and technological status and developed UAV systems to support precision agriculture. Interestingly, we did not find studies that employed empirical
methodologies or descriptive case studies, which constitutes a significant knowledge gap and calls for more research on this topic.
Co-citation analysis
According to Gmür (2006), co-citation analysis identifies similar publications and clusters them. Careful examination of a cluster can reveal a common field of research among the publications. We investigate the co-citation of the literature pertaining to agricultural drones to illustrate related subject areas and detect the intellectual patterns of publications. In this regard, Small (1973) recommended the use of cocitation analysis to study the most influential and seminal research
within a discipline. To limit the set to the most seminal articles (Goyal & Kumar, 2021), we set a co-citation threshold of 25, meaning that two articles must have been cited together in the reference lists of 25 or more different publications. The clustering was also conducted with a minimum cluster size 1 and without any method for merging smaller clusters with larger ones. As a result, six clusters were generated based on the similarity of studies and their intellectual structure. Table 6 shows the distribution of publications in each cluster.
Cluster 1: This cluster contains eighteen documents published after The publications in this cluster discuss the role of drones in supporting environmental monitoring, crop management, and weed management. For example, Manfreda et al. (2018) provide an overview of the current research and implementations of UAV in natural agricultural ecosystem monitoring and argue that the technology offers tremendous potential to drastically enhance environmental monitoring and reduce
the existing gap between field observation and conventional air and spaceborne remote sensing. This can be done by offering new capacity for improved temporal retrieval and spatial insights into large areas in an affordable way. UAVs can constantly sense the environment and send the resulting data to intelligent, centralized/ decentralized entities that control sensors to identify eventual problems, such as a lack of disease or water detection (Padua ´ et al., 2017). Adao ˜ et al. (2017) posit that UAVs are ideal for assessing plants’ conditions by capturing a vast volume of raw data related to water status, biomass estimation, and vigor assessment. UAV-mounted sensors could also be promptly deployed in proper environmental conditions to allow the timely capture of remote-sensing data (Von Bueren et al., 2015). By means of UAVs, farmers are able to carry out indoor farming activities by acquiring measurements from practically any place in the three-dimensional space of indoor farming environments (e.g., greenhouses), thereby ensuring local climate control and plant monitoring (Roldan ´ et al., 2015). In the context of precision
agriculture, crop management decisions necessitate accurate, reliable crop data with an appropriate temporal and spatial resolution (Gebbers & Adamchuk, 2010; Gevaert et al., 2015; Maes & Steppe, 2019). For this reason, Agüera Vega et al. (2015) used a UAV-mounted multispectral sensor system to acquire images of a sunflower crop during the growing season. Similarly, Huang et al. (2009) note that remote sensing based on UAVs could facilitate the measurement of crops and soil from the collected spectral data. Verger et al. (2014) developed and tested a technique for estimating a green area index (GAI) from UAV reflectance measurements in precision agriculture applications, focusing on wheat and rapeseed crops. Therefore, drones provide new possibilities for retrieving crop state information with frequent revisits and high spatial resolution (Dong et al., 2019; Garzonio et al., 2017; H. Zheng et al., 2016).
Clustering of influential publications on agricultural drones.
Cluster | Broad theme | References |
1 | Environmental monitoring, crop management, weed management | (Ad˜ ao et al., 2017; Agüera Vega et al., 2015; de Castro et al., 2018; Gomez-Cand ´ on ´ et al., 2014; Y. B. Huang et al., 2013; Khanal et al., 2017; Lopez-Granados, ´ 2011; Manfreda et al., 2018; P´ adua et al., 2017; Pena ˜ et al., 2013; P´erez-Ortiz et al., 2015; Rasmussen et al., 2013, 2016; Torres-S´ anchez et al., 2014; Torres-Sanchez, ´ Lopez-Granados, ´ & Pena, ˜ 2015; Verger et al., 2014; Von Bueren et al., 2015; C. Zhang & Kovacs, 2012) |
2 | Remote phenotyping, yield estimation, crop surface model, counting of plants | (Bendig et al., 2013, 2014; Geipel et al., 2014; Gnadinger ¨ & Schmidhalter, 2017; Haghighattalab et al., 2016; Holman et al., 2016; Jin et al., 2017; W. Li et al., 2016; Maimaitijiang et al., 2017; Sankaran et al., 2015; Schirrmann et al., 2016; Shi et al., 2016; Yue et al., 2017; X. Zhou et al., 2017) |
3 | Thermal imaging for water, multispectral imaging | (Baluja et al., 2012; Berni et al., 2009b; Berni et al., 2009a ; Candiago et al., 2015; Gago et al., 2015; Gonzalez-Dugo et al., 2013, 2014; Grenzdorffer ¨ et al., 2008; Khaliq et al., 2019; Matese et al., 2015; Ribeiro-Gomes et al., 2017; Santesteban et al., 2017; Uto et al., 2013) |
4 | Hypersectral imaging, spectral imaging | (Aasen et al., 2015a; Bareth et al., 2015; Hakala et al., 2013; Honkavaara et al., 2013a; Lucieer et al., 2014; Saari et al., 2011; Suomalainen et al., 2014) |
5 | 3D-Mapping Applications | (Jim´enez-Brenes et al., 2017; Nex & Remondino, 2014; Salamí et al., 2014; Torres-S´ anchez, Lopez- ´ Granados, Serrano, et al., 2015; Zahawi et al., 2015; Zarco-Tejada et al., 2014) |
6 | Agriculture surveillance | (S. R. Herwitz et al., 2004; Hunt et al., 2010; C. C. D. Lelong et al., 2008; Primicerio et al., 2012; Xiang & Tian, 2011) |
Furthermore, drones are useful for challenging tasks in agriculture, including weed mapping. Images captured by the devices have proven their usefulness for early weed detection in fields (de Castro et al., 2018; Jim´enez-Brenes et al., 2017; Lam et al., 2021; Lopez-Granados ´ et al., 2016; Rozenberg et al., 2021). In this regard, de Castro et al. (2018) posit that the merge of UAV imagery and Object-Based Image Analysis (OBIA) has enabled practitioners to overcome the issue of automating early detection in early season grassland crops, which is a big step forward in weed research. Likewise, Pena ˜ et al. (2013) point out that the use of ultra-high spatial resolution images from UAV in conjunction with an OBIA procedure makes it possible to generate weed maps in early maize crops that could be used in planning the implementation of in-season weed control measures, a task beyond the capability of satellite and traditional airborne images. Compared to image classification or object detection algorithms, semantic segmentation techniques are more effective at weed mapping tasks (J. Deng et al., 2020), thus enabling farmers to detect field conditions, mitigate losses, and improve yields throughout the growing season (Ramesh et al., 2020). Deep learningbased semantic segmentation can also provide an accurate measurement of vegetation cover from high-resolution aerial images (Ramesh et al., 2020; A. Zheng et al., 2022). Despite their potential for remote
sensing pixel classification, semantic segmentation techniques require significant computation and a prohibitively high GPU memory (J. Deng et al., 2020).
Based on machine learning and UAV, P´erez-Ortiz et al. (2015) suggested a weed mapping approach to provide site-specific weed control strategies when farmers adopt the early-post emergence weed control. Finally, Rasmussen et al. (2013) highlighted that drones provide inexpensive sensing with great spatial resolution flexibility. Overall, the publications in this cluster focus on exploring the potentials of UAVs to support remote sensing, crop monitoring, and weed mapping. Additional in-depth research is needed to further investigate how drone applications in environmental monitoring, crop management, and weed mapping can achieve more sustainable agriculture (Chamuah & Singh, 2019; Islam et al., 2021; Popescu et al., 2020; J. Su, Liu, et al., 2018) and address governance issues of this technology in crop insurance applications (Basnet & Bang, 2018; Chamuah & Singh, 2019, 2022; Meinen & Robinson, 2021). Researchers should concentrate on validating UAVcollected measurements with efficient processing techniques to enhance the ultimate quality of processed data (Manfreda et al., 2018). Furthermore, the development of appropriate algorithms that recognize pixels that display weeds in the digital images and eliminate irrelevant background during UAV weed mapping is needed (Gaˇsparovi´c et al., 2020; Hamylton et al., 2020; H. Huang et al., 2018, 2020; Lopez- ´ Granados et al., 2016). Additional research on the adoption of semantic segmentation techniques in plant recognition, leaf classification, and disease mapping is welcome (Fuentes-Pacheco et al., 2019; Kerkech et al., 2020).
Cluster 2. The publications in this cluster focused on several aspects of agricultural drones. Related to remote phenotyping, Sankaran et al. (2015) reviewed the potential of using low-altitude, high-resolution aerial imaging with UAVs for quick phenotyping of crops in the field, and they argue that, as compared to ground-based sensing platforms, small UAVs with adequate sensors offer several advantages, such as easier access to the field, highresolution data, efficient data collection,
rapid assessments of the field growth conditions, and low operational costs. However, the authors also note that the effective application of UAV for field phenotyping relies on two fundamental elements, namely, UAV features (e.g., safety, stability, positioning, autonomy) and sensor characteristics (e.g., resolution, weight, spectral wavelengths, field of view). Haghighattalab et al. (2016) proposed a semi-automated imageprocessing pipeline to retrieve plot-level data from UAV imagery and accelerate the breeding process. Holman et al. (2016) developed a high
throughput field phenotyping system and highlighted that UAV is able to collect quality, voluminous, field-based phenotypic data, and that the device is effective for large areas and across different field locations.
As yield estimation is an incredibly vital piece of information, particularly when being available on time, there is a potential for UAVs to provide all field measurements and efficiently acquire high-quality data (Daakir et al., 2017; Demir et al., 2018; Enciso et al., 2019; Kulbacki et al., 2018; Pudelko et al., 2012). In this regard, Jin et al. (2017) took advantage of the high resolution imagery obtained by UAVs at very low altitudes to develop and assess a method for estimating wheat plant density at the emergence stage. According to the authors, UAVs overcome the limitations of rover systems equipped with cameras and represent a non-invasive method to estimate plant density in crops, allowing farmers to achieve the high throughput necessary for field phenotyping independent of the trafficability of the soil. Li et al. (2016) collected hundreds of stereo images with extremely high resolution using a UAV-based system to estimate maize parameters, including canopy height and above-ground biomass. Finally, Yue et al. (2017) found that crop height determined from UAVs could enhance aboveground biomass (AGB) estimation.
An approach to monitor crop growth is the idea of developing crop surface models (Bendig et al., 2014, 2015; Holman et al., 2016; Panday, Shrestha, et al., 2020; Sumesh et al., 2021). Several studies highlighted the feasibility of images taken from UAV to capture plants’ height and monitor their growth. For example, Bendig et al. (2013) described the development of multi-temporal crop surface models with a very high resolution of less than 0.05 m using UAV. They aimed to detect crop
growth variability and its dependence on crop treatment, cultivar, and stress. Bendig et al. (2014) used UAVs to estimate fresh and dry biomass based on plant height extracted from crop surface models and found that, unlike airborne platforms and terrestrial laser scanning, the high resolution images from UAVs can significantly increase the accuracy of plant height modeling for different growth stages. In the same vein, Geipel et al. (2014) used UAVs in their research to acquire imagery
datasets for maize grain yield prediction at three different growth phases from early to mid-season and concluded that the combination of spectral and spatial modeling based on aerial images and crop surface models is a suitable method for predicting mid-season maize yield. Finally, Gnadinger ¨ and Schmidhalter (2017) examined the utility of UAV in precision phenotyping and highlighted that the use of this technology could enhance farm management and enable field experimentation for breeding and agronomic purposes. Overall, we observe that the publications in cluster 2 focus on the main advantages of UAVs in remote
phenotyping, yield estimation, crop surface modeling, and plant counting. Future studies can dig deeper by developing new methods for remote phenotyping that can automate and optimize the processing of remotely sensed data (Barabaschi et al., 2016; Liebisch et al., 2015; Mochida et al., 2015; S. Zhou et al., 2021). In addition, the performance of IoT sensors mounted on UAVs and the trade-off between their costs, labor, and precision of yield estimation need to be researched in the
future (Ju & Son, 2018a, 2018b; Xie & Yang, 2020; Yue et al., 2018). Ultimately, there is a need to develop efficient image processing methods that can generate reliable information, maximize efficiency in agricultural production, and minimize the manual counting work of the farmers (R. U. Khan et al., 2021; Koh et al., 2021; Lin & Guo, 2020; C. Zhang et al., 2020).
Cluster 3. The publications in this cluster discuss the different types of imaging systems for remote sensing of agriculture resources used on UAV platforms. In this regard, thermal imaging allows the monitoring of surface temperatures to prevent crop damage and detect drought stress early (Awais et al., 2022; García-Tejero et al., 2018; Sankaran et al., 2015; Santesteban et al., 2017; Yeom, 2021). Baluja et al. (2012) asserted that the use of multispectral and thermal cameras onboard the
UAV enabled researchers to obtain high resolution images and assess vine water status. This could be useful to develop novel water scheduling models using remote-sensing data (Baluja et al., 2012). Because of the
limited load capacity of UAVs, Ribeiro-Gomes et al. (2017) considered the integration of uncooled thermal cameras into UAVS to determine water stress in the plants, which makes this type of UAVs more efficient and viable than traditional satellite-based remote sensing and UAVs equipped with cooled thermal cameras. According to the authors, uncooled thermal cameras are lighter than cooled cameras, requiring appropriate calibration. Gonzalez-Dugo et al. (2014) showed that thermal imagery effectively generates spatial maps of crop water stress indices for assessing water status and quantifying water stress among and within citrus orchards. Gonzalez-Dugo et al. (2013) and Santesteban et al. (2017) investigated the use of high resolution UAV thermal imagery to estimate the water status variability of a commercial orchard and a vineyard.
Multispectral imaging could provide massive data in comparison with traditional RGB (Red, Green, and Blue) images (Ad˜ ao et al., 2017; Navia et al., 2016). This spectral data, along with spatial data, could aid in classification, mapping, forecasting, prediction, and detection purposes (Berni et al., 2009b). According to Candiago et al. (2015), UAVbased multispectral imaging could contribute massively to crop assessment and precise agriculture as a reliable and efficient resource. Also,
Khaliq et al. (2019) made a comparison between satellite and UAVbased multispectral imaging. The UAV-based images resulted in being more precise in describing vineyard variability as well as vigor maps for representing crop canopies. In a nutshell, articles in this cluster discuss the incorporation of thermal and multispectral imaging sensors into agricultural UAVs. Accordingly, more research is needed to understand how thermal and multispectral imaging can be integrated with AI
techniques (e.g., deep learning) to detect plant stress (Ampatzidis et al., 2020; Ampatzidis & Partel, 2019; Jung et al., 2021; Santesteban et al., 2017; Syeda et al., 2021). Such insights will help ensure more efficient and accurate detection as well as monitoring of plant growth, stress, and phenology (Buters et al., 2019; Cao et al., 2020; Neupane & BaysalGurel, 2021; L. Zhou et al., 2020).
Cluster 4. This cluster consists of seven papers that revolve around the crucial role of spectral imaging and hyperspectral imaging in supporting agriculture practices. Hyperspectral imaging has established itself as a remote-sensing method that enables quantitative assessment of the earth system (Schaepman et al., 2009).To be more precise, it enables the identification of surface materials, the quantification of (relative) concentrations, and the assignment of surface component proportions
within mixed pixels (Kirsch et al., 2018; Zhao et al., 2022). In other words, the higher spectral resolution provided by hyperspectral systems enables more accurate estimations of various parameters, such as vegetarian properties or leaf water content (Suomalainen et al., 2014). The researchers in this cluster investigated various aspects of such systems. Among others, Aasen et al. (2015b) offered a unique approach for deriving three-dimensional hyperspectral information from lightweight
snapshot cameras used on UAVs for vegetation monitoring. Lucieer et al. (2014) discussed the design, development, and aerial operations of a novel hyperspectral UAS as well as the calibration, analysis, and interpretation of the image data gathered with it. Finally, Honkavaara et al. (2013b) developed a comprehensive processing approach for FabryPerot interferometer-based spectral images and showed its use in a biomass estimate procedure for precision agriculture. Potential future pathways for this current cluster include emphasizing the need for technical improvements in sensor technologies (Aasen et al., 2015b) as well as the need for incorporating and enhancing complementary technologies, specifically big data and analytics (Ang & Seng, 2021; Radoglou-Grammatikis et al., 2020; Shakoor et al., 2019). The latter mainly stems from the ever-growing data generated by various sensors implemented in smart agriculture (C. Li & Niu, 2020; A. Rejeb et al., 2022; Y. Su & Wang, 2021).
Cluster 5. The publications in this cluster examined drones-based 3Dmapping applications. Using drones for 3D mapping could alleviate the complex fieldwork and increase efficiency substantially (Torres-Sanchez ´ et al., 2015). The five articles in the cluster mainly focused on plant monitoring applications. For example, to obtain three-dimensional data about canopy area, tree height, and crown volume, Torres-Sanchez ´ et al. (2015) used UAV technology to generate digital surface models and then object-based image analysis (OBIA) approaches. Further, Zarco-Tejada et al. (2014) quantified tree height by integrating UAV technology and three-dimensional photo-reconstruction methods. Jim´enez-Brenes Lopez-Granados, ´ De Castro, et al. (2017) demonstrated a new process for multi-temporal, 3D monitoring of dozens of olive trees by integrating UAV technology with advanced OBIA methodology. Interesting pathways for future works in this cluster include either improving current
methodologies (Zarco-Tejada et al., 2014) for digital surface modeling purposes (Ajayi et al., 2017; Jaud et al., 2016), such as OBIA (de Castro et al., 2018, 2020; Ventura et al., 2018), and photo reconstruction or developing novel methods (Díaz-Varela et al., 2015; Torres-S´ anchez et al., 2015).
Cluster 6. This cluster discusses the role of drones in agricultural surveillance. UAVs could complement and overcome the shortcomings of satellite and aircraft imaging. For example, they could provide high resolution near real-time imaging with less fuel or piloting challenges, resulting in constant and real-time surveillance and improvements in decision-making (S. Herwitz et al., 2004). Another key contribution of UAVs is their ability to provide site-specific data for precision agriculture or site-specific farming as their high resolution, detailed data about various parameters enable farmers to divide the land into homogeneous parts and treat them accordingly (Hunt et al., 2010; C. C. Lelong et al., 2008; Primicerio et al., 2012). Such UAV-based agricultural surveillance can support food security monitoring and decision making (S. R. Herwitz et al., 2004). To advance research in agricultural surveillance, not only improvements in sensors, UAVs, and other related technologies and their communication and data transfer methods are needed (Ewing et al., 2020; Shuai et al., 2019), but also integrating drones with various technologies for optimizing different tasks in relation to smart agriculture, such as monitoring, agricultural surveillance, and decisionmaking, is a high potential research area (Alsamhi et al., 2021; Popescu et al., 2020; Vuran et al., 2018). In this regard, IoT, WSNs, and big data offer interesting complementary capabilities (van der Merwe et al., 2020). Implementation costs, cost savings, energy efficiency, and data security are among the under-researched areas for such integration (Masroor et al., 2021).
Countries and academic institutions
The final step included the investigation of the country of origin and the academic affiliations of the authors. Through this analysis, we aim to better understand the geographic distribution of scholars who contribute to the applications of drones in agriculture. It is noteworthy to notice the diversity of countries and academic institutions. From a country perspective, the USA, China, India, and Italy rank at the top of the list in terms of the number of publications (Table 7). The current
research on agricultural drones is largely centered in North American and Asian countries, mainly due to their high engagement in precision agriculture applications. For example, in the USA, the market of agricultural drones was estimated at 841.9 million USD in the year 2020, accounting for approximately 30% of the global market share (ReportLinker, 2021). Ranking as the world’s largest economy, China is predicted to reach an approximate market size of 2.6 billion USD in the year 2027. This country is appealing for agricultural drones to overcome productivity issues and achieve better yields, labor alleviation, and lesser production inputs. However, the adoption of the technology in China is also driven by factors such as the population size and the needto innovate and improve existing crop management practices.
Top most productive countries and universities/organizations that contribute to
agricultural drone-related research.
Rank | Countries |
1 | USA |
2 | China |
3 | India |
4 | Italy |
5 | Spain |
6 | Germany |
7 | Brazil |
8 | Australia |
9 | Japan |
10 | United Kingdom |
Rank | Universities/ Organizations |
1 | Chinese Academy of Sciences |
2 | Ministry of Agriculture of the People’s Republic of China |
3 | Consejo Superior de Investigaciones Científicas |
4 | Texas A&M University |
5 | China Agricultural University |
6 | USDA Agricultural Research Service |
7 | CSIC – Instituto de Agricultura Sostenible IAS |
8 | Purdue University |
9 | Consiglio Nazionale delle Ricerche |
10 | South China Agricultural University |
From a university and organizational perspective, Chinese Academy of Sciences tops the list in terms of the number of publications, followed by Ministry of Agriculture of the People’s Republic of China and Consejo Superior de Investigaciones Científicas. Chinese Academy of Sciences is represented by the authors Liao Xiaohan and Li Jun; Han Wenting represents the Ministry of Agriculture of the People’s Republic of China; and Consejo Superior de Investigaciones Científicas is represented by Lopez-Granados, ´ F. and Pena, ˜ Jos´e María S. From the USA, universities like the Texas A&M University and Purdue University find their
mention. The universities with the highest number of publications and their connections are shown in Fig. 4. Additionally, this list includes institutions such as the Consiglio Nazionale delle Ricerche and the Consejo Superior de Investigaciones Científicas that are active in scientific research, but are not academic institutions.
Our selection included a wide variety of journals, encompassing virtually all of the available data. As is shown in Table 8, Remote Sensing with 258 articles ranks at the top, followed by Journal of Intelligent and Robotic Systems: Theory and Applications with 126 and Computers and Electronics in Agriculture with 98 articles. While Remote Sensing is mostly focused on the application and development of drones, Computers and Electronics in Agriculture mainly covers advances in computer hardware, software, electronics, and control systems in agriculture. Cross-area outlets, such as IEEE Robotics and Automation Letters with 87 publications and IEEE Access with 34 publications, are also premier outlets in the field. The top fifteen outlets have contributed to the literature with 959 documents, which is approximately 20.40% of all publications. A journal co-citation analysis enables us to examine the importance and similarity between publications. The co-citation analysis yields three clusters, as shown in Fig. 5. The red cluster consists of journals such as Remote Sensing, Computer and Electronics in Agriculture, Sensors,
and the International Journal of Remote Sensing. All of these outlets are highly reputable journals in the areas of remote sensing and precision agriculture. The green cluster contains journals that deal with robotics, such as Journal of Intelligent and Robotic Systems: Theory and Applications, IEEE Robotics and Automation Letters, IEEE Access, and Drones. These outlets mostly publish papers on automation and are useful for agricultural engineers. The final cluster is formed by journals related to agronomy and agricultural engineering, like Agronomy and International Journal of Agricultural and Biological Engineering.
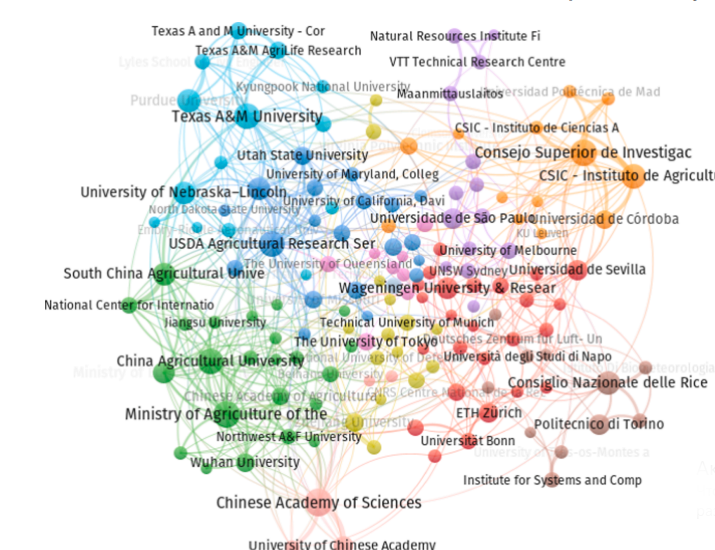
Top 15 journals in agricultural drone-related research.
Rank | Journal | Count |
1 | Remote Sensing | 258 |
2 | Journal of Intelligent and Robotic Systems: Theory and Applications | 126 |
3 | Computers and Electronics in Agriculture | 98 |
4 | IEEE Robotics and Automation Letters | 87 |
5 | Sensors | 73 |
6 | International Journal of Remote Sensing | 42 |
7 | Precision Agriculture | 41 |
8 | Drones | 40 |
9 | Agronomy | 34 |
10 | IEEE Access | 34 |
11 | International Journal of Advanced Robotic Systems | 31 |
12 | International Journal of Agricultural and Biological Engineering | 25 |
13 | PLoS ONE | 25 |
14 | Journal of Field Robotics | 23 |
15 | Biosystems Engineering | 23 |
Conclusion
Summary
In this study, we summarized and analyzed existing research on agricultural drones. Applying various bibliometric techniques, we strived to obtain a better understanding of the intellectual structure of agricultural drone-related research. In sum, our review offers several contributions by identifying and discussing keywords in the literature, revealing knowledge clusters while forming semantically similar communities in the field of drones, outlining earlier research, and suggesting future research directions. Below, we outline the main findings of the review on the development of agricultural drones:
• The overall literature has grown rapidly and attracted enormous attention over the last decade, as indicated by the rise in the number of articles after 2012. Even though this knowledge field is yet to attain its full maturity (Barrientos et al., 2011; Maes & Steppe, 2019), several questions are still unanswered. For instance, the utility of drones in indoor farming is still open for debate (Aslan et al., 2022; Krul et al., 2021; Rold´ an et al., 2015). The complexity of fields scenes and the different imaging circumstances (e.g., shadows and illumination) could result in a higher spectral in-class variance (Yao et al., 2019). Even in the later research phases, researchers have been challenged to determine optimal flight plans according to particular scenarios and required image quality (Soares et al., 2021; Tu et al.,
2020).
• We notice that the field has progressed from developing efficient UAV systems to incorporating AI techniques, such as machine learning and deep learning in the design of agricultural drones (Bah et al., 2018; Kitano et al., 2019; Maimaitijiang et al., 2020; Mazzia et al., 2020; Tetila et al., 2020).
• Research on agricultural drones predominately discussed remote sensing by exploring the potentials of the technology in environmental monitoring, crop management, and weed management (cluster 1) as well as remote phenotyping and yield estimation (cluster 2). A set of influential studies on agricultural drones include Austin (2010), Berni et al. (2009)a, Herwitz et al. (2004), Nex and Remondino (2014), and Zhang and Kovacs (2012). These studies developed the conceptual basis of drone-related research in the context of agriculture.
• Related to the methodology, we observed that most of the research done so far had been composed of either system design, conceptual, or review-based studies (Inoue, 2020; Nex & Remondino, 2014; P´erez-Ortiz et al., 2015; Yao et al., 2019). We also notice a lack of empirical, qualitative, and case-study-based methods at work in investigating agricultural drones.
• Recently, topics related to precision agriculture, AI techniques, precision viticulture, and water stress assessment have drawn substantial attention (Espinoza et al., 2017; Gomez-Cand ´ on ´ et al., 2016; Matese et al., 2015; Matese & Di Gennaro, 2018, 2021; Z. Zhou et al., 2021). Careful examination of research clusters in two separate eras, 1990–2010 and 2011–2021, reveals the progress of the domain’s intellectual structure. The period from 1990 to 2010 constituted the build-up of central notions and the concepts of drones, which is obvious from the discussion of UAV design, development, and implementation. In the second era, the research focus expands on prior studies, making an effort to synthesize UAV use cases in agriculture. We also found numerous studies that discuss drone applications in imaging tasks and precision agriculture.
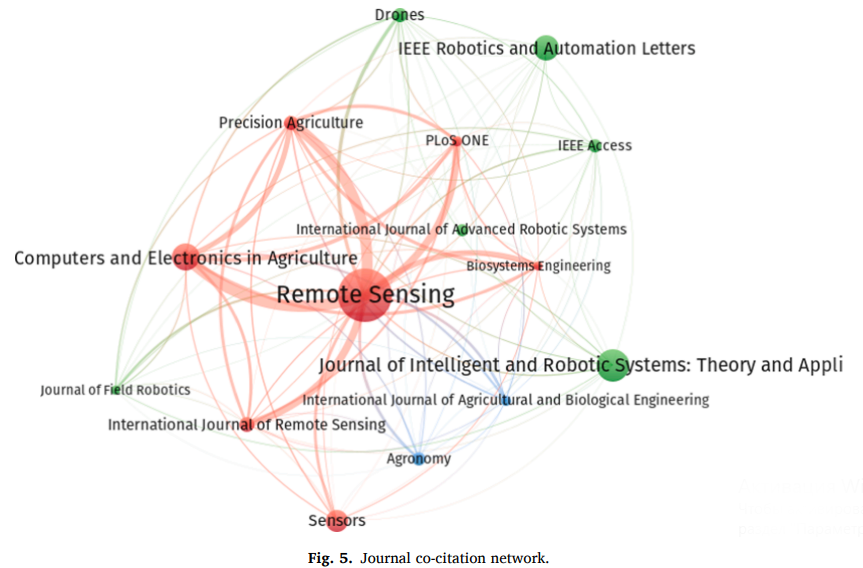
Rank | Journal | Count |
1 | Remote Sensing | 258 |
2 | Journal of Intelligent and Robotic Systems: Theory and | 126 |
Applications | ||
3 | Computers and Electronics in Agriculture | 98 |
4 | IEEE Robotics and Automation Letters | 87 |
5 | Sensors | 73 |
6 | International Journal of Remote Sensing | 42 |
7 | Precision Agriculture | 41 |
8 | Drones | 40 |
9 | Agronomy | 34 |
10 | IEEE Access | 34 |
11 | International Journal of Advanced Robotic Systems | 31 |
12 | International Journal of Agricultural and Biological Engineering | 25 |
13 | PLoS ONE | 25 |
14 | Journal of Field Robotics | 23 |
15 | Biosystems Engineering | 22 |
Implications
Our bibliometric review was designed and conducted with scholars, farmers, agricultural experts, crop consultants, and UAV system designers in mind. To the authors’ best knowledge, this is one of the first original reviews that has undertaken an in-depth bibliometric analysis of
drone applications in agriculture. We have conducted a comprehensive review of this knowledge body, employing citation and co-citation analyses of publications. Our attempts to describe the intellectual structure of drone research also offer new insights for academics. A careful review of the keywords used over time reveals the hotspots and focal research areas in the drone-related literature. Furthermore, we present a list of most cited studies to identify the most impactful research works completed in the field. The identification of articles and keywords could consequently provide a solid starting point to uncover several avenues for future studies.
Importantly, we revealed clusters that classify comparable works and elaborated on the results. The studies classified in clusters aid in understanding the intellectual structure of UAV research. Notably, we discovered a dearth of studies that investigate drones’ adoption factors
and barriers in farming activities (see Table 9). Future researchers could address this potential gap by conducting empirical investigations that evaluate drones’ adoption factors in different farming activities and climatic conditions. Furthermore, case study-based research regarding the effectiveness of drones should be backed with real data from the field. Also, involving farmers and managers in academic research would be advantageous for both the theoretical and practical advancement of drone research. We were also able to identify the most prominent researchers and their contributions, which is valuable because awareness of recent seminal works can offer some guidance for future academic endeavors.
Table 9
UAV adoption barriers.
Barrier | Description |
Data security | Cyber security is a major challenge for implementing IoT solutions (Masroor et al., 2021). |
Interoperability and integration | Various technologies such as UAV, WSN, IoT, etc. should be integrated and transmit data which increase the complexity level (Alsamhi et al., 2021; Popescu et al., 2020; Vuran et al., 2018). |
Implementation costs | This is specifically the case for small farmers and for integrating various cutting-edge technologies ( Masroor et al., 2021). |
Labor knowledge and expertise | Skilled drone pilots are needed to operate UAVs. Also, implementing various cutting-edge technologies requires skilled workers (Y. B. Huang et al., 2013; Tsouros et al., 2019). |
Engine Power and flight duration | Drones cannot be operated for long hours and cover large areas (Hardin & Hardin, 2010; Laliberte et al., 2007). |
Stability, reliability, and maneuverability | Drones are not stable during bad weather conditions (Hardin & Hardin, 2010; Laliberte et al., 2007). |
Payload limitations and sensors’ quality | Drones only can carry limited loads leads to capability of loading lower quality sensors (Nebiker et al., 2008). |
Regulation | As drones can be dangerous as well, there are severe regulations in some areas (Hardin & Jensen, 2011; Laliberte & Rango, 2011). |
Farmers’ knowledge and interest | As other cutting-edge technologies, the drones’ successful implementation needs expertise and also accompanied with uncertainties (Fisher et al., 2009; Lambert et al., 2004; Stafford, 2000). |
Since there is a constant need to efficiently use available resources to maximize yields, farmers can take advantage of drones to ensure rapid, accurate, and cost-effective scanning of their fields. The technology can support farmers to determine their crops’ condition and assess water status, the stage of ripening, insect infestations, and nutritional needs. The remote-sensing capabilities of drones can provide farmers with crucial data to anticipate issues at an early stage and promptly make suitable interventions. However, the benefits of the technology can only be realized if the challenges are properly addressed. In light of the
current problems regarding data security, sensor technology issues (e.g., the reliability or accuracy of measurements), complexity of integration, and substantial implementation costs, future studies must also examine the technical, economic, and operational feasibility of integrating agricultural drones and other cutting-edge technologies.
Limitations
Our study has several limitations. Firstly, the findings are determined by the publications selected for the final analysis. It is challenging to capture all relevant studies related to agricultural drones, particularly those not indexed in the Scopus database. Further, the data collection process is limited to the setting of search keywords, which may not be inclusive and lead to inconclusive findings. Thus, future studies need to pay more attention to the underlying issue of data collection to make
more reliable conclusions. Another limitation concerns new publications with a low number of citations. The bibliometric analysis is biased toward earlier publications as they tend to receive more citations over the years. Recent studies need a certain amount of time to attract attention and accumulate citations. Consequently, recent studies that bring a paradigm shift would not rank in the top ten influential works. This limitation is prevalent in the examination of rapidly emerging research domains like agricultural drones. As we have consulted Scopus to study the literature for this work, future researchers could consider different
databases, such as the Web of Science and IEEE Xplore, to expand the horizon and enhance the research structure.
Potential bibliometric studies may consider other vital knowledge sources like conference papers, chapters, and books to generate novel insights. Despite mapping and investigating global publications on agricultural drones, our findings did not reveal the reasons behind the scholarly outputs of universities. This paves the way to a novel area of research in qualitatively explaining why some universities are more productive than others when it comes to research about agricultural
drones. In addition, future studies could provide insights into the potential of drones to increase farming sustainability in several ways such as environmental monitoring, crop management, and weed mapping as indicated by several researchers (Chamuah & Singh, 2019; Islam et al., 2021; Popescu et al., 2020; J. Su, Liu, et al., 2018b). Since the textual analysis was not possible due to the high number of selected papers, there is a need for systematic literature reviews that examine the
research methods used and the involvement of farmers in prior studies. In short, our analysis of drone research exposes the invisible linkages of this knowledge body. This review therefore helps to uncover the relationships among publications and explores the intellectual structure of the research field. It also depicts the linkages between the various aspects of the literature, such as authors’ keywords, affiliations, and countries.
Declaration of Competing Interest
The authors declare that they have no known competing financial interests or personal relationships that could have appeared to influence the work reported in this paper.
Appendix 1
TITLE-ABS-KEY (((drone* OR “unmanned aerial vehicle” OR uav* OR “unmanned aircraft system” OR uas OR “remotely piloted aircraft”) AND (agricultural OR agriculture OR farming OR farmer))) AND (EXCLUDE (PUBYEAR, 2022)) AND (LIMIT-TO (LANGUAGE, “English”)).
References
Aasen, H., Burkart, A., Bolten, A., Bareth, G., 2015. Generating 3D hyperspectral information with lightweight UAV snapshot cameras for vegetation monitoring: from
camera calibration to quality assurance. ISPRS J. Photogramm. Remote Sens. 108, 245–259. https://doi.org/10.1016/j.isprsjprs.2015.08.002.
Abd-Elrahman, A., Pearlstine, L., Percival, F., 2005. Development of pattern recognition algorithm for automatic bird detection from unmanned aerial vehicle imagery.
Survey. Land Inform. Sci. 65 (1), 37–45.
Abdollahi, A., Rejeb, K., Rejeb, A., Mostafa, M.M., Zailani, S., 2021. Wireless sensor networks in agriculture: insights from bibliometric analysis. Sustainability 13 (21),
12011.
Aboutalebi, M., Torres-Rua, A.F., Kustas, W.P., Nieto, H., Coopmans, C., McKee, M.,Assessment of different methods for shadow detection in high-resolution optical imagery and evaluation of shadow impact on calculation of NDVI, and evapotranspiration. Irrig. Sci. 37 (3), 407–429. https://doi.org/10.1007/s00271-018-0613-9.
Adao, ˜ T., Hruˇska, J., Padua, ´ L., Bessa, J., Peres, E., Morais, R., Sousa, J.J., 2017. Hyperspectral imaging: a review on UAV-based sensors, data processing and
applications for agriculture and forestry. Remote Sensing 9 (11). https://doi.org/ 10.3390/rs9111110.
Agüera Vega, F., Ramírez, F.C., Saiz, M.P., Rosúa, F.O., 2015. Multi-temporal imaging using an unmanned aerial vehicle for monitoring a sunflower crop. Biosyst. Eng.
132, 19–27. https://doi.org/10.1016/j.biosystemseng.2015.01.008.
Ajayi, O.G., Salubi, A.A., Angbas, A.F., Odigure, M.G., 2017. Generation of accurate digital elevation models from UAV acquired low percentage overlapping images. Int.
J. Remote Sens. 38 (8–10), 3113–3134. https://doi.org/10.1080/ 01431161.2017.1285085.
Ali, I., Greifeneder, F., Stamenkovic, J., Neumann, M., Notarnicola, C., 2015. Review of machine learning approaches for biomass and soil moisture retrievals from remote sensing data. Remote Sensing 7 (12), 16398–16421.
Alsamhi, S.H., Afghah, F., Sahal, R., Hawbani, A., Al-qaness, M.A.A., Lee, B., Guizani, M., Green internet of things using UAVs in B5G networks: A review of applications
and strategies. Ad. Hoc. Netw. 117, 102505 https://doi.org/10.1016/j. adhoc.2021.102505.
Al-Thani, N., Albuainain, A., Alnaimi, F., Zorba, N., 2020. Drones for Sheep Livestock Monitoring. In: 20th IEEE Mediterranean Electrotechnical Conference. https://doi.
org/10.1109/MELECON48756.2020.9140588.
Ampatzidis, Y., Partel, V., 2019. UAV-based high throughput phenotyping in citrus utilizing multispectral imaging and artificial intelligence. Remote Sensing 11 (4), https://doi.org/10.3390/rs11040410.
Ampatzidis, Y., Partel, V., Costa, L., 2020. Agroview: Cloud-based application to process, analyze and visualize UAV-collected data for precision agriculture applications utilizing artificial intelligence. Comput. Electron. Agric. 174, 105457 https://doi. org/10.1016/j.compag.2020.105457.
Ang, K.-L.-M., Seng, J.K.P., 2021. Big data and machine learning with hyperspectral information in agriculture. IEEE Access 9, 36699–36718. https://doi.org/10.1109/
ACCESS.2021.3051196.
Aquilani, C., Confessore, A., Bozzi, R., Sirtori, F., Pugliese, C., 2022. Review: precision Livestock Farming technologies in pasture-based livestock systems. Animal 16 (1), https://doi.org/10.1016/j.animal.2021.100429.
Armenta-Medina, D., Ramirez-Delreal, T.A., Villanueva-Vasquez, ´ D., Mejia-Aguirre, C., Trends on advanced information and communication technologies for
improving agricultural productivities: a bibliometric analysis. Agronomy 10 (12), Article 12. https://doi.org/10.3390/agronomy10121989.
Armstrong, I., Pirrone-Brusse, M., Smith, A., Jadud, M., 2011. The flying gator: towards aerial robotics in occam-π. Commun. Process Architect. 2011, 329–340. https://doi. org/10.3233/978-1-60750-774-1-329.
Arora, S.D., Chakraborty, A., 2021. Intellectual structure of consumer complaining behavior (CCB) research: A bibliometric analysis. J. Business Res. 122, 60–74.
Aslan, M.F., Durdu, A., Sabanci, K., Ropelewska, E., Gültekin, S.S., 2022.
A comprehensive survey of the recent studies with UAV for precision agriculture in open fields and greenhouses. Appl. Sci. 12 (3), 1047. https://doi.org/10.3390/
app12031047.
Atkinson, J. A., Jackson, R. J., Bentley, A. R., Ober, E., & Wells, D. M. (2018). Field Phenotyping for the Future. In Annual Plant Reviews online (pp. 719–736). John
Wiley & Sons, Ltd. doi: 10.1002/9781119312994.apr0651.
Austin, R., 2010. Unmanned Aircraft Systems: UAVS Design, Development and Deployment. In: Unmanned Aircraft Systems: UAVS Design, Development and
Deployment. John Wiley and Sons. https://doi.org/10.1002/9780470664797.
Awais, M., Li, W., Cheema, M.J.M., Zaman, Q.U., Shaheen, A., Aslam, B., Zhu, W., Ajmal, M., Faheem, M., Hussain, S., Nadeem, A.A., Afzal, M.M., Liu, C., 2022. UAVbased remote sensing in plant stress imagine using high-resolution thermal sensor for digital agriculture practices: a meta-review. Int. J. Environ. Sci. Technol. https://doi.
org/10.1007/s13762-021-03801-5.
Bacco, M., Berton, A., Ferro, E., Gennaro, C., Gotta, A., Matteoli, S., Paonessa, F., Ruggeri, M., Virone, G., Zanella, A., 2018. Smart farming: Opportunities, challenges
and technology enablers. 2018 IoT Vertical and. Topical Summit on Agriculture -Tuscany (IOT Tuscany) 1–6. https://doi.org/10.1109/IOTTUSCANY.2018.8373043.
Bah, M.D., Hafiane, A., Canals, R., 2018. Deep learning with unsupervised data labeling for weed detection in line crops in UAV images. Remote Sensing 10 (11), 1690.
https://doi.org/10.3390/rs10111690.
Baldi, S., 1998. Normative versus social constructivist processes in the allocation of citations: a network-analytic model. Am. Sociol. Rev. 63 (6), 829–846. https://doi.
org/10.2307/2657504.
Baluja, J., Diago, M.P., Balda, P., Zorer, R., Meggio, F., Morales, F., Tardaguila, J., 2012. Assessment of vineyard water status variability by thermal and multispectral
imagery using an unmanned aerial vehicle (UAV). Irrig. Sci. 30 (6), 511–522. https://doi.org/10.1007/s00271-012-0382-9.
Barabaschi, D., Tondelli, A., Desiderio, F., Volante, A., Vaccino, P., Val`e, G., Cattivelli, L.,Next generation breeding. Plant Sci. 242, 3–13. https://doi.org/10.1016/j.
plantsci.2015.07.010.
Barbedo, J.G.A., Koenigkan, L.V., 2018. Perspectives on the use of unmanned aerial systems to monitor cattle. Outlook Agric. 47 (3), 214–222. https://doi.org/10.1177/0030727018781876.
Bareth, G., Aasen, H., Bendig, J., Gnyp, M.L., Bolten, A., Jung, A., Michels, R., Soukkamaki, ¨ J., 2015. Low-weight and UAV-based hyperspectral full-frame cameras
for monitoring crops: Spectral comparison with portable spectroradiometer measurements. Photogrammetrie, Fernerkundung, Geoinformation 2015 (1), 69–79.
https://doi.org/10.1127/pfg/2015/0256.
Barrientos, A., Colorado, J., del Cerro, J., Martinez, A., Rossi, C., Sanz, D., Valente, J., Aerial remote sensing in agriculture: A practical approach to area coverage
and path planning for fleets of mini aerial robots. J. Field Rob. 28 (5), 667–689. https://doi.org/10.1002/rob.20403.
Basiri, A., Mariani, V., Silano, G., Aatif, M., Iannelli, L., Glielmo, L., 2022. A survey on the application of path-planning algorithms for multi-rotor UAVs in precision
agriculture. J. Navig. 75 (2), 364–383.
Basnet, B., Bang, J., 2018. The state-of-the-art of knowledge-intensive agriculture: a review on applied sensing systems and data analytics. J. Sens. 2018, 1–13.
Bendig, J., Bolten, A., Bareth, G., 2013. UAV-based imaging for multi-temporal, very high resolution crop surface models to monitor crop growth variability. Photogrammetrie, Fernerkundung, Geoinformation 2013 (6), 551–562. https://doi. org/10.1127/1432-8364/2013/0200.
Bendig, J., Bolten, A., Bennertz, S., Broscheit, J., Eichfuss, S., Bareth, G., 2014. Estimating biomass of barley using crop surface models (CSMs) derived from UAVbased RGB imaging. Remote Sensing 6 (11), 10395–10412.
Bendig, J., Yu, K., Aasen, H., Bolten, A., Bennertz, S., Broscheit, J., Gnyp, M.L., Bareth, G., 2015. Combining UAV-based plant height from crop surface models,
visible, and near infrared vegetation indices for biomass monitoring in barley. Int. J. Appl. Earth Obs. Geoinf. 39, 79–87. https://doi.org/10.1016/j.jag.2015.02.012.
Berni, J.A., Zarco-Tejada, P.J., Sepulcre-Canto, ´ G., Fereres, E., Villalobos, F., 2009a. Mapping canopy conductance and CWSI in olive orchards using high resolution
thermal remote sensing imagery. Remote Sens. Environ. 113 (11), 2380–2388. https://doi.org/10.1016/j.rse.2009.06.018.
Berni, J.A., Zarco-Tejada, P.J., Suarez, ´ L., Fereres, E., 2009b. Thermal and narrowband multispectral remote sensing for vegetation monitoring from an unmanned aerial vehicle. IEEE Trans. Geosci. Remote Sens. 47 (3), 722–738.
Bouzembrak, Y., Klüche, M., Gavai, A., Marvin, H.J.P., 2019. Internet of Things in food safety: Literature review and a bibliometric analysis. Trends Food Sci. Technol. 94,54–64. https://doi.org/10.1016/j.tifs.2019.11.002.
Brewster, C., Roussaki, I., Kalatzis, N., Doolin, K., Ellis, K., 2017. IoT in agriculture: Designing a Europe-wide large-scale pilot. IEEE Commun. Mag. 55 (9), 26–33.
Buters, T.M., Belton, D., Cross, A.T., 2019. Multi-sensor UAV tracking of individual seedlings and seedling communities at millimetre accuracy. Drones 3 (4), 81.
https://doi.org/10.3390/drones3040081.
Candiago, S., Remondino, F., De Giglio, M., Dubbini, M., Gattelli, M., 2015. Evaluating multispectral images and vegetation indices for precision farming applications from UAV images. Remote Sensing 7 (4), 4026–4047. https://doi.org/10.3390/rs70404026.
Cao, Y., Li, G.L., Luo, Y.K., Pan, Q., Zhang, S.Y., 2020. Monitoring of sugar beet growth indicators using wide-dynamic-range vegetation index (WDRVI) derived from UAV
multispectral images. Comput. Electron. Agric. 171, 105331 https://doi.org/10.1016/j.compag.2020.105331.
Casillas, J., Acedo, F., 2007. Evolution of the intellectual structure of family business literature: a bibliometric study of FBR. Family Business Rev. 20 (2), 141–162.
Cen, H., Wan, L., Zhu, J., Li, Y., Li, X., Zhu, Y., Weng, H., Wu, W., Yin, W., Xu, C., Bao, Y., Feng, L., Shou, J., He, Y., 2019. Dynamic monitoring of biomass of rice under
different nitrogen treatments using a lightweight UAV with dual image-frame snapshot cameras. Plant Methods 15 (1), 32. https://doi.org/10.1186/s13007-019-
0418-8.
Chamuah, A., Singh, R., 2019. Securing sustainability in Indian agriculture through civilian UAV: a responsible innovation perspective. SN Appl. Sci. 2 (1), 106. https://
doi.org/10.1007/s42452-019-1901-6.
Chamuah, A., Singh, R., 2022. Responsible governance of civilian unmanned aerial vehicle (UAV) innovations for Indian crop insurance applications. J. Responsible
Technol. 9, 100025 https://doi.org/10.1016/j.jrt.2022.100025.
Chen, A., Orlov-Levin, V., Meron, M., 2019. Applying high-resolution visible-channel aerial imaging of crop canopy to precision irrigation management. Agric. Water
Manag. 216, 196–205. https://doi.org/10.1016/j.agwat.2019.02.017.
Daakir, M., Pierrot-Deseilligny, M., Bosser, P., Pichard, F., Thom, C., Rabot, Y., Martin, O., 2017. Lightweight UAV with on-board photogrammetry and singlefrequency GPS positioning for metrology applications. ISPRS J. Photogramm. Remote Sens. 127, 115–126. https://doi.org/10.1016/j.isprsjprs.2016.12.007.
Dawaliby, S., Aberkane, A., Bradai, A., 2020. Blockchain-based IoT platform for autonomous drone operations management. In: Proceedings of the 2nd ACM
MobiCom Workshop on Drone Assisted Wireless Communications for 5G and Beyond, pp. 31–36. https://doi.org/10.1145/3414045.3415939.
Day, R.A., Gastel, B., 1998. How to write and publish a scientific paper. Cambridge University Press. de Castro, A.I., Pena, ˜ J.M., Torres-Sanchez, ´ J., Jim´enez-Brenes, F.M., ValenciaGredilla, F., Recasens, J., Lopez-Granados, ´ F., 2020. Mapping cynodon dactylon infesting cover crops with an automatic decision tree-OBIA procedure and UAV imagery for precision viticulture. Remote Sensing 12 (1), 56. https://doi.org/10.3390/rs12010056.
de Castro, A.I., Torres-S´ anchez, J., Pena, ˜ J.M., Jim´enez-Brenes, F.M., Csillik, O., Lopez- ´Granados, F., 2018. An automatic random forest-OBIA algorithm for early weed mapping between and within crop rows using UAV imagery. Remote Sensing 10 (2). https://doi.org/10.3390/rs10020285.
Demir, N., Sonmez, ¨ N.K., Akar, T., Ünal, S., 2018. Automated Measurement of Plant Height of Wheat Genotypes Using a DSM Derived from UAV Imagery. Proceedings 2 (7), 350. https://doi.org/10.3390/ecrs-2-05163.
Deng, J., Zhong, Z., Huang, H., Lan, Y., Han, Y., Zhang, Y., 2020. Lightweight semantic segmentation network for real-time weed mapping using unmanned aerial vehicles. Appl. Sci. 10 (20), 7132. https://doi.org/10.3390/app10207132.
Deng, L., Mao, Z., Li, X., Hu, Z., Duan, F., Yan, Y., 2018. UAV-based multispectral remote sensing for precision agriculture: a comparison between different cameras. ISPRS J. Photogramm. Remote Sens. 146, 124–136.
Diaz-Gonzalez, F.A., Vuelvas, J., Correa, C.A., Vallejo, V.E., Patino, D., 2022. Machine learning and remote sensing techniques applied to estimate soil indicators – review. Ecol. Ind. 135, 108517 https://doi.org/10.1016/j.ecolind.2021.108517.
Díaz-Varela, R.A., De la Rosa, R., Leon, ´ L., Zarco-Tejada, P.J., 2015. High-resolution airborne UAV imagery to assess olive tree crown parameters using 3D photo
reconstruction: application in breeding trials. Remote Sensing 7 (4), 4213–4232. https://doi.org/10.3390/rs70404213.
Dixit, A., Jakhar, S.K., 2021. Airport capacity management: a review and bibliometric analysis. J. Air Transp. Manag. 91, 102010.
Dong, T., Shang, J., Liu, J., Qian, B., Jing, Q., Ma, B., Huffman, T., Geng, X., Sow, A., Shi, Y., Canisius, F., Jiao, X., Kovacs, J.M., Walters, D., Cable, J., Wilson, J., 2019.
Using RapidEye imagery to identify within-field variability of crop growth and yield in Ontario, Canada. Precision Agric. 20 (6), 1231–1250. https://doi.org/10.1007/
s11119-019-09646-w.
Dutta, P.K., Mitra, S., 2021. Application of agricultural drones and iot to understand food supply chain during post COVID-19. In: Choudhury, A., Biswas, A., Prateek, M.,
Chakrabarti, A. (Eds.), Agricultural Informatics: Automation Using the IoT and Machine Learning. Wiley, pp. 67–87. van Eck, N., Waltman, L., 2009. Software survey: VOSviewer, a computer program for bibliometric mapping. Scientometrics 84 (2), 523–538. https://doi.org/10.1007/s11192-009-0146-3.
Elijah, O., Rahman, T.A., Orikumhi, I., Leow, C.Y., Hindia, M.N., 2018. An overview of Internet of Things (IoT) and data analytics in agriculture: benefits and challenges.
IEEE Internet Things J. 5 (5), 3758–3773.
Enciso, J., Avila, C.A., Jung, J., Elsayed-Farag, S., Chang, A., Yeom, J., Landivar, J.,Maeda, M., Chavez, J.C., 2019. Validation of agronomic UAV and field
measurements for tomato varieties. Comput. Electron. Agric. 158, 278–283. https:// doi.org/10.1016/j.compag.2019.02.011.
Espinoza, C.Z., Khot, L.R., Sankaran, S., Jacoby, P.W., 2017. High resolution multispectral and thermal remote sensing-based water stress assessment in
subsurface irrigated grapevines. Remote Sensing 9 (9), 961. https://doi.org/ 10.3390/rs9090961.
Ewing, J., Oommen, T., Jayakumar, P., Alger, R., 2020. Utilizing hyperspectral remote sensing for soil gradation. Remote Sensing 12 (20), 3312. ttps://doi.org/10.3390/
rs12203312.
Fawcett, D., Panigada, C., Tagliabue, G., Boschetti, M., Celesti, M., Evdokimov, A., Biriukova, K., Colombo, R., Miglietta, F., Rascher, U., Anderson, K., 2020. Multiscale evaluation of drone-based multispectral surface reflectance and vegetation indices in operational conditions. Remote Sensing 12 (3), 514.
Feng, X., Yan, F., Liu, X., 2019. Study of wireless communication technologies on Internet of Things for precision agriculture. Wireless Pers. Commun. 108 (3),
1785–1802.
Ferreira, M.P., Pinto, C.F., Serra, F.R., 2014. The transaction costs theory in international business research: a bibliometric study over three decades. Scientometrics 98 (3), 1899–1922. https://doi.org/10.1007/s11192-013-1172-8.
Fisher, P., Abuzar, M., Rab, M., Best, F., Chandra, S., 2009. Advances in precision agriculture in south-eastern Australia. I. a regression methodology to simulate
spatial variation in cereal yields using farmers’ historical paddock yields and normalised difference vegetation index. Crop Pasture Sci. 60 (9), 844–858.
Floreano, D., Wood, R.J., 2015. Science, technology and the future of small autonomous drones. Nature 521 (7553), 460–466. https://doi.org/10.1038/nature14542.
Friha, O., Ferrag, M.A., Shu, L., Maglaras, L.A., Wang, X., 2021. Internet of things for the future of smart agriculture: a comprehensive survey of emerging technologies. IEEE CAA J. Autom. Sinica 8 (4), 718–752.
Fuentes-Pacheco, J., Torres-Olivares, J., Roman-Rangel, E., Cervantes, S., JuarezLopez, P., Hermosillo-Valadez, J., Rendon-Mancha, ´ J.M., 2019. Fig plant segmentation from aerial images using a deep convolutional encoder-decoder network. Remote Sensing 11 (10), 1157. https://doi.org/10.3390/rs11101157.
Gago, J., Douthe, C., Coopman, R.E., Gallego, P.P., Ribas-Carbo, M., Flexas, J., Escalona, J., Medrano, H., 2015. UAVs challenge to assess water stress for
sustainable agriculture. Agric. Water Manag. 153, 9–19. https://doi.org/10.1016/j. agwat.2015.01.020.
García-Tejero, I.F., Rubio, A.E., Vinuela, ˜ I., Hern´ andez, A., Guti´errez-Gordillo, S., Rodríguez-Pleguezuelo, C.R., Dur´ an-Zuazo, V.H., 2018. Thermal imaging at plant
level to assess the crop-water status in almond trees (cv. Guara) under deficit irrigation strategies. Agric. Water Manag. 208, 176–186. https://doi.org/10.1016/j.
agwat.2018.06.002.
Garzonio, R., Di Mauro, B., Colombo, R., Cogliati, S., 2017. Surface reflectance and suninduced fluorescence spectroscopy measurements using a small hyperspectral UAS. Remote Sensing 9 (5), 472. https://doi.org/10.3390/rs9050472. Gaˇsparovi´c, M., Zrinjski, M., Barkovi´c, Đ., Radoˇcaj, D., 2020. An automatic method for
weed mapping in oat fields based on UAV imagery. Comput. Electron. Agric.
Gebbers, R., Adamchuk, V.I., 2010. Precision agriculture and food security. Science 327 (5967), 828–831. https://doi.org/10.1126/science.1183899.
Geipel, J., Link, J., Claupein, W., 2014. Combined spectral and spatial modeling of corn yield based on aerial images and crop surface models acquired with an unmanned aircraft system. Remote Sensing 6 (11), 10335–10355. https://doi.org/10.3390/rs61110335.
Geng, D., Feng, Y., Zhu, Q., 2020. Sustainable design for users: a literature review and bibliometric analysis. Environ. Sci. Pollut. Res. 27 (24), 29824–29836. https://doi. org/10.1007/s11356-020-09283-1.
Gevaert, C.M., Suomalainen, J., Tang, J., Kooistra, L., 2015. Generation of spectraltemporal response surfaces by combining multispectral satellite and hyperspectral
UAV imagery for precision agriculture applications. IEEE J. Sel. Top. Appl. Earth Obs. Remote Sens. 8 (6), 3140–3146. ttps://doi.org/10.1109/JSTARS.2015.2406339.
Gill, S.S., Chana, I., Buyya, R., 2017. IoT based agriculture as a cloud and big dataservice: the beginning of digital India. J. Org. and End User Comput. (JOEUC) 29 (4),
1–23.
Gmür, M., 2006. Co-citation analysis and the search for invisible colleges: a methodological evaluation. Scientometrics 57 (1), 27–57. https://doi.org/10.1023/
a:1023619503005.
Gnadinger, ¨ F., Schmidhalter, U., 2017. Digital counts of maize plants by Unmanned Aerial Vehicles (UAVs). Remote Sensing 9 (6). Https://doi.org/10.3390/rs9060544.
Gokto ¨ ǧan, A.H., Sukkarieh, S., Bryson, M., Randle, J., Lupton, T., Hung, C., 2010. A Rotary-wing unmanned air vehicle for aquatic weed surveillance and
management. J. Intell. Robotic Syst.: Theor. Appl. 57 (1–4), 467–484. https://doi. org/10.1007/s10846-009-9371-5.
Gomez-Cand ´ on, ´ D., De Castro, A.I., Lopez-Granados, ´ F., 2014. Assessing the accuracy of mosaics from unmanned aerial vehicle (UAV) imagery for precision agriculture purposes in wheat. Precis. Agric. 15 (1), 44–56. https://doi.org/10.1007/s11119-013-9335-4.
Gomez-Cand ´ on, ´ D., Virlet, N., Labb´e, S., Jolivot, A., Regnard, J.-L., 2016. Field phenotyping of water stress at tree scale by UAV-sensed imagery: new insights for
thermal acquisition and calibration. Precis. Agric. 17 (6), 786–800. https://doi.org/10.1007/s11119-016-9449-6.
Gonzalez-Dugo, V., Zarco-Tejada, P.J., Fereres, E., 2014. Applicability and limitations of using the crop water stress index as an indicator of water deficits in citrus orchards. Agric. For. Meteorol. 198–199, 94–104. https://doi.org/10.1016/j. agrformet.2014.08.003.
Gonzalez-Dugo, V., Zarco-Tejada, P., Nicolas, ´ E., Nortes, P.A., Alarcon, ´ J.J., Intrigliolo, D.S., Fereres, E., 2013. Using high resolution UAV thermal imagery to
assess the variability in the water status of five fruit tree species within a commercial orchard. Precis. Agric. 14 (6), 660–678. https://doi.org/10.1007/s11119-013-9322-9.
Goyal, K., Kumar, S., 2021. Financial literacy: A systematic review and bibliometric analysis. Int. J. Consumer Studies 45 (1), 80–105. https://doi.org/10.1111/
ijcs.12605.
Grenzdorffer, ¨ G.J., Engel, A., Teichert, B., 2008. The photogrammetric potential of lowcost uavs in forestry and agriculture. International Archives of the Photogrammetry, Remote Sensing and Spatial Information Sciences – ISPRS Archives 37, 1207–1213. https://www.scopus.com/inward/record.uri?eid=2-s2.0-85039543258&partnerI D=40&md5=b4b2d639257e8ddb5a373d15959c4e1e.
Guan, S., Fukami, K., Matsunaka, H., Okami, M., Tanaka, R., Nakano, H., Sakai, T., Nakano, K., Ohdan, H., Takahashi, K., 2019. Assessing correlation of high-resolution
NDVI with fertilizer application level and yield of rice and wheat crops using small UAVs. Remote Sensing 11 (2), 112.
Gundolf, K., Filser, M., 2013. Management research and religion: a citation analysis. J. Bus. Ethics 112 (1), 177–185.
Guo, Q., Zhu, Y., Tang, Y., Hou, C., He, Y., Zhuang, J., Zheng, Y., Luo, S., 2020. CFD simulation and experimental verification of the spatial and temporal distributions of
the downwash airflow of a quad-rotor agricultural UAV in hover. Comput. Electron. Agric. 172, 105343 https://doi.org/10.1016/j.compag.2020.105343.
Haghighattalab, A., Gonz´ alez P´erez, L., Mondal, S., Singh, D., Schinstock, D., Rutkoski, J., Ortiz-Monasterio, I., Singh, R.P., Goodin, D., Poland, J., 2016.
Application of unmanned aerial systems for high throughput phenotyping of large wheat breeding nurseries. Plant Methods 12 (1). https://doi.org/10.1186/s13007-
016-0134-6.
Hakala, T., Honkavaara, E., Saari, H., Makynen, ¨ J., Kaivosoja, J., Pesonen, L., & Pol ¨ onen, ¨I., 2013. Spectral imaging from UAVs under varying illumination conditions. In G. G. Bill R. (Ed.), International Archives of the Photogrammetry, Remote Sensing and Spatial Information Sciences—ISPRS Archives (Vol. 40, Issue 1W2, pp. 189–194). International Society for Photogrammetry and Remote Sensing. https://www.scopus.com/inward/record.uri?eid=2-s2.0-848875632.
Hamylton, S.M., Morris, R.H., Carvalho, R.C., Roder, N., Barlow, P., Mills, K., Wang, L.Evaluating techniques for mapping island vegetation from unmanned aerial
vehicle (UAV) images: Pixel classification, visual interpretation and machine learning approaches. Int. J. Appl. Earth Obs. Geoinf. 89, 102085 https://doi.org/
10.1016/j.jag.2020.102085.
Haque, A., Islam, N., Samrat, N.H., Dey, S., Ray, B., 2021. Smart farming through responsible leadership in bangladesh: possibilities, opportunities, and beyond.
Sustainability 13 (8), 4511.
Hardin, P.J., Hardin, T.J., 2010. Small-scale remotely piloted vehicles in environmental research. Geography Compass 4 (9), 1297–1311. ttps://doi.org/10.1111/j.1749-
8198.2010.00381.x.
Hardin, P.J., Jensen, R.R., 2011. Small-scale unmanned aerial vehicles in environmental remote sensing: challenges and opportunities. GISci. Remote Sens. 48 (1), 99–111. https://doi.org/10.2747/1548-1603.48.1.99.
He, Y., Nie, P., Zhang, Q., Liu, F., 2021. Agricultural Internet of Things: technologies and applications, (1st ed. 2021 edition). Springer.
Herwitz, S.R., Johnson, L.F., Dunagan, S.E., Higgins, R.G., Sullivan, D.V., Zheng, J., Lobitz, B.M., Leung, J.G., Gallmeyer, B.A., Aoyagi, M., Slye, R.E., Brass, J.A., 2004.
Imaging from an unmanned aerial vehicle: agricultural surveillance and decision support. Comput. Electron. Agric. 44 (1), 49–61. https://doi.org/10.1016/j.
compag.2004.02.006.
Holman, F.H., Riche, A.B., Michalski, A., Castle, M., Wooster, M.J., Hawkesford, M.J., High throughput field phenotyping of wheat plant height and growth rate in field plot trials using UAV based remote sensing. Remote Sensing 8 (12). https://doi. org/10.3390/rs8121031.
Honkavaara, E., Saari, H., Kaivosoja, J., Pol ¨ onen, ¨ I., Hakala, T., Litkey, P., M¨akynen, J., Pesonen, L., 2013. Processing and assessment of spectrometric, stereoscopic imagery collected using a lightweight UAV spectral camera for precision agriculture. Remote Sensing 5 (10), 5006–5039. https://doi.org/10.3390/rs5105006.
Hossein Motlagh, N., Taleb, T., Arouk, O., 2016. Low-altitude unmanned aerial vehiclesbased internet of things services: comprehensive survey and future perspectives. IEEE Internet Things J. 3 (6), 899–922. https://doi.org/10.1109/JIOT.2016.2612119.
Hrabar, S., Sukhatme, G.S., Corke, P., Usher, K., Roberts, J., 2005. Combined optic-flow and stereo-based navigation of urban canyons for a UAV. In: 2005 IEEE/RSJ
International Conference on Intelligent Robots and Systems, pp. 3309–3316. https://doi.org/10.1109/IROS.2005.1544998.
Hsu, T.-C., Yang, H., Chung, Y.-C., Hsu, C.-H., 2020. A Creative IoT agriculture platform for cloud fog computing. Sustain. Comput. Inf. Syst. 28, 100285.
Huang, H., Deng, J., Lan, Y., Yang, A., Deng, X., Zhang, L., Gonzalez-Andujar, J.L., 2018. A fully convolutional network for weed mapping of unmanned aerial vehicle (UAV) imagery. PLoS ONE 13 (4), e0196302.
Huang, H., Lan, Y., Yang, A., Zhang, Y., Wen, S., Deng, J., 2020. Deep learning versus Object-based Image Analysis (OBIA) in weed mapping of UAV imagery. Int. J.
Remote Sens. 41 (9), 3446–3479. https://doi.org/10.1080/01431161.2019.1706112.
Huang, H., Yang, A., Tang, Y., Zhuang, J., Hou, C., Tan, Z., Dananjayan, S., He, Y., Guo, Q., Luo, S., 2021. Deep color calibration for UAV imagery in crop monitoring
using semantic style transfer with local to global attention. Int. J. Appl. Earth Obs. Geoinf. 104, 102590 https://doi.org/10.1016/j.jag.2021.102590.
Huang, Y.B., Thomson, S.J., Hoffmann, W.C., Lan, Y.B., Fritz, B.K., 2013. Development and prospect of unmanned aerial vehicle technologies for agricultural production
management. Int. J. Agric. Biol. Eng. 6 (3), 1–10. https://doi.org/10.3965/j. ijabe.20130603.001.
Huang, Y., Hoffmann, W.C., Lan, Y., Wu, W., Fritz, B.K., 2009. Development of a spray system for an unmanned aerial vehicle platform. Appl. Eng. Agric. 25 (6), 803–809.
Hunt Jr., E.R., Dean Hively, W., Fujikawa, S.J., Linden, D.S., Daughtry, C.S.T., McCarty, G.W., 2010. Acquisition of NIR-green-blue digital photographs from
unmanned aircraft for crop monitoring. Remote Sensing 2 (1), 290–305. https://doi. org/10.3390/rs2010290. Inoue, Y., 2020. Satellite- and drone-based remote sensing of crops and soils for smart farming–a review. Soil Sci. Plant Nutr. 66 (6), 798–810. https://doi.org/10.1080/00380768.2020.1738899.
Islam, N., Rashid, M.M., Pasandideh, F., Ray, B., Moore, S., Kadel, R., 2021. A review of applications and communication technologies for Internet of Things (IoT) and
Unmanned Aerial Vehicle (UAV) based sustainable smart farming. Sustainability 13 (4), 1821. https://doi.org/10.3390/su13041821.
Jaud, M., Passot, S., Le Bivic, R., Delacourt, C., Grandjean, P., Le Dantec, N., 2016. Assessing the accuracy of high resolution digital surface models computed by
PhotoScan® and MicMac® in sub-optimal survey conditions. Remote Sensing 8 (6), https://doi.org/10.3390/rs8060465.
Jim´enez-Brenes, F.M., Lopez-Granados, ´ F., Castro, A.I., Torres-S´ anchez, J., Serrano, N., Pena, ˜ J.M., 2017. Quantifying pruning impacts on olive tree architecture and annual canopy growth by using UAV-based 3D modelling. Plant Methods 13 (1). https://doi.org/10.1186/s13007-017-0205-3.
Jin, X., Liu, S., Baret, F., Hemerl´e, M., Comar, A., 2017. Estimates of plant density of wheat crops at emergence from very low altitude UAV imagery. Remote Sens.
Environ. 198, 105–114. https://doi.org/10.1016/j.rse.2017.06.007.
Jinbo, C., Xiangliang, C., Han-Chi, F., Lam, A., 2019. Agricultural product monitoring system supported by cloud computing. Cluster Comput. 22 (4), 8929–8938.
Ju, C., & Son, H. I. 2018a. Performance evaluation of multiple UAV systems for remote sensing in agriculture. Proceedings of the Workshop on Robotic Vision and Action in Agriculture at the IEEE International Conference on Robotics and Automation (ICRA), Brisbane, Australia, 21–26.
Ju, C., Son, H.I., 2018b. Multiple UAV systems for agricultural applications: control, implementation, and evaluation. Electronics 7 (9), 162. https://doi.org/10.3390/
electronics7090162.
Jung, J., Maeda, M., Chang, A., Bhandari, M., Ashapure, A., Landivar-Bowles, J., 2021. The potential of remote sensing and artificial intelligence as tools to improve the
resilience of agriculture production systems. Curr. Opin. Biotechnol. 70, 15–22. https://doi.org/10.1016/j.copbio.2020.09.003.
Kalischuk, M., Paret, M.L., Freeman, J.H., Raj, D., Da Silva, S., Eubanks, S., Wiggins, D.J., Lollar, M., Marois, J.J., Mellinger, H.C., Das, J., 2019. An improved crop scouting technique incorporating unmanned aerial vehicle–assisted multispectral crop imaging into conventional scouting practice for gummy stem blight in watermelon. Plant Dis. 103 (7), 1642–1650.
Kapoor, K.K., Tamilmani, K., Rana, N.P., Patil, P., Dwivedi, Y.K., Nerur, S., 2018. Advances in social media research: past, present and future. Inform. Syst. Front. 20
(3), 531–558.
Kerkech, M., Hafiane, A., Canals, R., 2020. VddNet: vine disease detection network based on multispectral images and depth map. Remote Sensing 12 (20), 3305. https://doi. org/10.3390/rs12203305.
Khaliq, A., Comba, L., Biglia, A., Ricauda Aimonino, D., Chiaberge, M., Gay, P., 2019. Comparison of satellite and UAV-based multispectral imagery for vineyard
variability assessment. Remote Sensing 11 (4). https://doi.org/10.3390/rs11040436.
Khan, P.W., Byun, Y.-C., Park, N., 2020. IoT-blockchain enabled optimized provenance system for food industry 4.0 using advanced deep learning. Sensors 20 (10), 2990.
Khan, R.U., Khan, K., Albattah, W., Qamar, A.M., Ullah, F., 2021. Image-based detection of plant diseases: from classical machine learning to deep learning journey. Wireless Commun. Mobile Comput. 2021, 1–13.
Khan, S., Tufail, M., Khan, M.T., Khan, Z.A., Iqbal, J., Alam, M., Le, K.N.Q., 2021. A novel semi-supervised framework for UAV based crop/weed classification. PLoS ONE 16 (5), e0251008.
Khanal, S., Fulton, J., Shearer, S., 2017. An overview of current and potential applications of thermal remote sensing in precision agriculture. Comput. Electron.
Agric. 139, 22–32. https://doi.org/10.1016/j.compag.2017.05.001.
Khanna, A., Kaur, S., 2019. Evolution of Internet of Things (IoT) and its significant impact in the field of Precision Agriculture. Comput. Electron. Agric. 157, 218–231.
Kim, W., Khan, G.F., Wood, J., Mahmood, M.T., 2016. Employee engagement for sustainable organizations: keyword analysis using social network analysis and burst
detection approach. Sustainability 8 (7), 631.
Kirsch, M., Lorenz, S., Zimmermann, R., Tusa, L., Mockel, ¨ R., Hodl, ¨ P., Booysen, R., Khodadadzadeh, M., Gloaguen, R., 2018. Integration of terrestrial and drone-borne
hyperspectral and photogrammetric sensing methods for exploration mapping and mining monitoring. Remote Sensing 10 (9), 1366. https://doi.org/10.3390/
rs10091366.
Kitano, B.T., Mendes, C.C.T., Geus, A.R., Oliveira, H.C., Souza, J.R., 2019. Corn plant counting using deep learning and UAV images. IEEE Geosci. Remote Sens. Lett. 1–5 https://doi.org/10.1109/LGRS.2019.2930549.
Koh, J.C.O., Spangenberg, G., Kant, S., 2021. Automated machine learning for highthroughput image-based plant phenotyping. Remote Sensing 13 (5), 858. https://
doi.org/10.3390/rs13050858.
Kovalev, I.V., Voroshilova, A.A., 2020. Modern technological trends in the development of the ecosystem of cargo UAVs. J. Phys. Conf. Ser. 1515 (5), 052068 https://doi. org/10.1088/1742-6596/1515/5/052068.
Krul, S., Pantos, C., Frangulea, M., Valente, J., 2021. Visual SLAM for indoor livestock and farming using a small drone with a monocular camera: a feasibility study.
Drones 5 (2), 41. https://doi.org/10.3390/drones5020041.
Kulbacki, M., Segen, J., Knie´c, W., Klempous, R., Kluwak, K., Nikodem, J., Kulbacka, J., Serester, A., 2018. Survey of drones for agriculture automation from planting to
harvest. In: INES 2018 – IEEE 22nd International Conference on Intelligent Engineering Systems, pp. 000353–358. https://doi.org/10.1109/INES.2018.8523943.
Lagkas, T., Argyriou, V., Bibi, S., Sarigiannidis, P., 2018. UAV IoT framework views and challenges: towards protecting drones as “Things”. Sensors 18 (11), 4015. https://doi.org/10.3390/s18114015.
Laliberte, A.S., Rango, A., 2011. Image processing and classification procedures for analysis of sub-decimeter imagery acquired with an unmanned aircraft over arid
rangelands. GISci. Remote Sens. 48 (1), 4–23. https://doi.org/10.2747/1548-1603.48.1.4.
Laliberte, A.S., Rango, A., Herrick, J.E., 2007. Unmanned aerial vehicles for rangeland mapping and monitoring: a comparison of two systems. ASPRS Annual Conference Proceedings.
Lam, O.H.Y., Dogotari, M., Prüm, M., Vithlani, H.N., Roers, C., Melville, B., Zimmer, F., Becker, R., 2021. An open source workflow for weed mapping in native grassland
using unmanned aerial vehicle: Using Rumex obtusifolius as a case study. Eur. J.Remote Sens. 54 (sup1), 71–88. https://doi.org/10.1080/22797254.2020.1793687.
Lambert, D.M., Lowenberg-DeBoer, J., Griffin, T.W., Peone, J., Payne, T., Daberkow, S.G., 2004. Adoption, profitability, and making better use of precision farming data.
Working paper. Purdue University. https://doi.org/10.22004/ag.econ.28615.
Lelong, C.C.D., Burger, P., Jubelin, G., Roux, B., Labb´e, S., Baret, F., 2008. Assessment of unmanned aerial vehicles imagery for quantitative monitoring of wheat crop in small plots. Sensors 8 (5), 3557–3585. https://doi.org/10.3390/s8053557.
Li, C., Niu, B., 2020. Design of smart agriculture based on big data and Internet of things. Int. J. Distrib. Sens. Netw. 16 (5) ttps://doi.org/10.1177/1550147720917065.
Li, W., Niu, Z., Chen, H., Li, D., Wu, M., Zhao, W., 2016. Remote estimation of canopy height and aboveground biomass of maize using high-resolution stereo images from a low-cost unmanned aerial vehicle system. Ecol. Ind. 67, 637–648. https://doi.org/10.1016/j.ecolind.2016.03.036.
Liakos, K.G., Busato, P., Moshou, D., Pearson, S., Bochtis, D., 2018. Machine learning in agriculture: a review. Sensors 18 (8), 2674.
Liebisch, F., Kirchgessner, N., Schneider, D., Walter, A., Hund, A., 2015. Remote, aerial phenotyping of maize traits with a mobile multi-sensor approach. Plant Methods 11 (1), 9. https://doi.org/10.1186/s13007-015-0048-8.
Lin, Z., Guo, W., 2020. Sorghum panicle detection and counting using unmanned aerial system images and deep learning. Front. Plant Sci. 11.
Liu, S., Guo, L., Webb, H., Ya, X., Chang, X., 2019. Internet of Things monitoring system of modern eco-agriculture based on cloud computing. IEEE Access 7, 37050–37058.
Lopez-Granados, ´ F., 2011. Weed detection for site-specific weed management: mapping and real-time approaches. Weed Res. 51 (1), 1–11. https://doi.org/10.1111/j.1365-3180.2010.00829.x.
Lopez-Granados, ´ F., Torres-Sanchez, ´ J., De Castro, A.-I., Serrano-P´erez, A., MesasCarrascosa, F.-J., Pena, ˜ J.-M., 2016. Object-based early monitoring of a grass weed in a grass crop using high resolution UAV imagery. Agron. Sustain. Dev. 36 (4), 1–12
Lopez-Granados, ´ F., Torres-S´ anchez, J., Serrano-P´erez, A., de Castro, A.I., MesasCarrascosa, F.-J., Pena, ˜ J.-M., 2016. Early season weed mapping in sunflower using UAV technology: variability of herbicide treatment maps against weed thresholds. Precis. Agric. 17 (2), 183–199.
Lucieer, A., Malenovský, Z., Veness, T., Wallace, L., 2014. HyperUAS – imaging spectroscopy from a multirotor unmanned aircraft system. J. Field Rob. 31 (4),
571–590. https://doi.org/10.1002/rob.21508.
Lumme, J., Karjalainen, M., Kaartinen, H., Kukko, A., Hyyppa, ¨ J., Hyypp¨ a, H., Jaakkola, A., & Kleemola, J., 2008. Terrestrial laser scanning of agricultural crops. In J. J.
Chen J. Maas H–G. (Ed.), International Archives of the Photogrammetry, Remote Sensing and Spatial Information Sciences—ISPRS Archives (Vol. 37, pp. 563–566).
International Society for Photogrammetry and Remote Sensing. https://www.scopus .com/inward/record.uri?eid=2-s2.0-84919356328&partnerID=40&md5=574
b802131a99d16318ce619a01ca1bf.
Ma, L., Li, M., Ma, X., Cheng, L., Du, P., Liu, Y., 2017. A review of supervised objectbased land-cover image classification. ISPRS J. Photogramm. Remote Sens. 130,
277–293. https://doi.org/10.1016/j.isprsjprs.2017.06.001.
Maes, W.H., Steppe, K., 2019. Perspectives for remote sensing with unmanned aerial vehicles in precision agriculture. Trends Plant Sci. 24 (2), 152–164. https://doi.org/10.1016/j.tplants.2018.11.007.
Maimaitijiang, M., Ghulam, A., Sidike, P., Hartling, S., Maimaitiyiming, M., Peterson, K., Shavers, E., Fishman, J., Peterson, J., Kadam, S., Burken, J., Fritschi, F., 2017.
Unmanned aerial system (UAS)-based phenotyping of soybean using multi-sensor data fusion and extreme learning machine. ISPRS J. Photogramm. Remote Sens. 134, 43–58. https://doi.org/10.1016/j.isprsjprs.2017.10.011. Maimaitijiang, M., Sagan, V., Sidike, P., Daloye, A.M., Erkbol, H., Fritschi, F.B., 2020.
Crop monitoring using satellite/UAV data fusion and machine learning. Remote Sensing 12 (9), 1357. https://doi.org/10.3390/rs12091357.
Manfreda, S., McCabe, M., Miller, P., Lucas, R., Pajuelo Madrigal, V., Mallinis, G., Ben Dor, E., Helman, D., Estes, L., Ciraolo, G., Müllerova, ´ J., Tauro, F., de Lima, M., de
Lima, J., Maltese, A., Frances, F., Caylor, K., Kohv, M., Perks, M., Ruiz-P´erez, G., Su, Z., Vico, G., Toth, B., 2018. On the use of unmanned aerial systems for
environmental monitoring. Remote Sensing 10 (4), 641.
Marinko, R.A., 1998. Citations to women’s studies journals in dissertations, 1989 and The Serials Librarian 35 (1–2), 29–44. https://doi.org/10.1300/J123v35n01_
03.
Masroor, R., Naeem, M., Ejaz, W., 2021. Resource management in UAV-assisted wireless networks: an optimization perspective. Ad Hoc Netw. 121, 102596 https://doi.org/10.1016/j.adhoc.2021.102596.
Matese, A., Di Gennaro, S.F., 2018. Practical applications of a multisensor UAV platform based on multispectral, thermal and RGB high resolution images in precision
viticulture. Agriculture 8 (7), 116. https://doi.org/10.3390/agriculture8070116.
Matese, A., Di Gennaro, S.F., 2021. Beyond the traditional NDVI index as a key factor to mainstream the use of UAV in precision viticulture. Sci. Rep. 11 (1), 2721. https://doi.org/10.1038/s41598-021-81652-3.
Matese, A., Toscano, P., Di Gennaro, S.F., Genesio, L., Vaccari, F.P., Primicerio, J., Belli, C., Zaldei, A., Bianconi, R., Gioli, B., 2015. Intercomparison of UAV, aircraft
and satellite remote sensing platforms for precision viticulture. Remote Sensing 7 (3), 2971–2990. https://doi.org/10.3390/rs70302971.
Mazzia, V., Comba, L., Khaliq, A., Chiaberge, M., Gay, P., 2020. UAV and machine learning based refinement of a satellite-driven vegetation index for precision
agriculture. Sensors 20 (9), 2530. https://doi.org/10.3390/s20092530.
McCain, K.W., 1990. Mapping authors in intellectual space: a technical overview. J. Am. Soc. Info. Sci. 41 (6), 433–443.
Meinen, B.U., Robinson, D.T., 2021. Agricultural erosion modelling: evaluating USLE and WEPP field-scale erosion estimates using UAV time-series data. Environ. Modell. Software 137, 104962. https://doi.org/10.1016/j.envsoft.2021.104962.
Melville, B., Lucieer, A., Aryal, J., 2019. Classification of lowland native grassland communities using hyperspectral Unmanned Aircraft System (UAS) Imagery in the
Tasmanian midlands. Drones 3 (1), 5.
Messina, G., Modica, G., 2020. Applications of UAV thermal imagery in precision agriculture: state of the art and future research outlook. Remote Sensing 12 (9), https://doi.org/10.3390/rs12091491.
Mishra, D., Luo, Z., Jiang, S., Papadopoulos, T., Dubey, R., 2017. A bibliographic study on big data: concepts, trends and challenges. Business Process Manag. J. 23 (3),
555–573.
Mochida, K., Saisho, D., Hirayama, T., 2015. Crop improvement using life cycle datasets acquired under field conditions. Front. Plant Sci. 6 https://doi.org/10.3389/
fpls.2015.00740.
Mogili, UM.R., Deepak, B.B.V.L., 2018. Review on application of drone systems in precision agriculture. Procedia Comput. Sci. 133, 502–509.
Moharana, S., Dutta, S., 2016. Spatial variability of chlorophyll and nitrogen content of rice from hyperspectral imagery. ISPRS J. Photogramm. Remote Sens. 122, 17–29.
Muangprathub, J., Boonnam, N., Kajornkasirat, S., Lekbangpong, N., Wanichsombat, A.,
Nillaor, P., 2019. IoT and agriculture data analysis for smart farm. Comput. Electron. Agric. 156, 467–474.
Nansen, C., Elliott, N., 2016. Remote sensing and reflectance profiling in entomology. Annu. Rev. Entomol. 61 (1), 139–158. https://doi.org/10.1146/annurev-ento010715-023834.
Navia, J., Mondragon, I., Patino, D., Colorado, J., 2016. Multispectral mapping in agriculture: terrain mosaic using an autonomous quadcopter UAV. Int. Conf.
Unmanned Aircraft Syst. (ICUAS) 2016, 1351–1358. https://doi.org/10.1109/ ICUAS.2016.7502606.
Nayyar, A., Nguyen, B.-L., Nguyen, N.G., 2020. The internet of drone things (Iodt): future envision of smart drones. Adv. Intell. Syst. Comput. 1045, 563–580. https://doi.org/10.1007/978-981-15-0029-9_45.
Nebiker, S., Annen, A., Scherrer, M., Oesch, D., 2008. A light-weight multispectral sensor for micro UAV—opportunities for very high resolution airborne remote sensing. Int. Arch. Photogramm. Remote Sens. Spat. Inf. Sci 37 (B1), 1193–1200.
Negash, L., Kim, H.-Y., Choi, H.-L., 2019. Emerging UAV applications in agriculture. In: 2019 7th International Conference on Robot Intelligence Technology and
Applications (RiTA), pp. 254–257. https://doi.org/10.1109/RITAPP.2019.8932853.
Nerur, S.P., Rasheed, A.A., Natarajan, V., 2008. The intellectual structure of the strategic management field: an author co-citation analysis. Strateg. Manag. J. 29 (3),
319–336.
Neupane, K., Baysal-Gurel, F., 2021. Automatic identification and monitoring of plant diseases using unmanned aerial vehicles: a review. Remote Sensing 13 (19), 3841. https://doi.org/10.3390/rs13193841.
Nex, F., Remondino, F., 2014. UAV for 3D mapping applications: a review. Appl. Geomatics 6 (1), 1–15. https://doi.org/10.1007/s12518-013-0120-x.
Niu, H., Hollenbeck, D., Zhao, T., Wang, D., Chen, Y., 2020. Evapotranspiration estimation with small UAVs in precision agriculture. Sensors 20 (22), 6427. https://
doi.org/10.3390/s20226427.
Osareh, F., 1996. Bibliometrics, Citation Analysis and Co-Citation Analysis. A Review of Literature I 46 (3), 149–158. https://doi.org/10.1515/libr.1996.46.3.149.
P´ adua, L., Vanko, J., Hruˇska, J., Ad˜ ao, T., Sousa, J.J., Peres, E., Morais, R., 2017. UAS, sensors, and data processing in agroforestry: a review towards practical applications. Int. J. Remote Sens. 38 (8–10), 2349–2391. https://doi.org/10.1080/01431161.2017.1297548.
Panday, U.S., Pratihast, A.K., Aryal, J., Kayastha, R.B., 2020. A review on drone-based data solutions for cereal crops. Drones 4 (3), 1–29. https://doi.org/10.3390/
drones4030041.
Parsaeian, M., Shahabi, M., Hassanpour, H., 2020. Estimating oil and protein content of sesame seeds using image processing and artificial neural network. J. Am. Oil
Chemists’ Soc. 97 (7), 691–702.
Pena, ˜ J.M., Torres-S´anchez, J., de Castro, A.I., Kelly, M., Lopez-Granados, ´ F., Suarez, O., Weed mapping in early-season maize fields using object-based analysis of
unmanned aerial vehicle (UAV) Images. PLoS ONE 8 (10), e77151.
P´erez-Ortiz, M., Pena, ˜ J.M., Guti´errez, P.A., Torres-S´ anchez, J., Herv´ as-Martínez, C.,
Lopez-Granados, ´ F., 2015. A semi-supervised system for weed mapping in sunflower crops using unmanned aerial vehicles and a crop row detection method. Appl. Soft Comput. J. 37, 533–544. https://doi.org/10.1016/j.asoc.2015.08.027.
Pincheira, M., Vecchio, M., Giaffreda, R., Kanhere, S.S., 2021. Cost-effective IoT devices as trustworthy data sources for a blockchain-based water management system in precision agriculture. Comput. Electron. Agric. 180, 105889.
Popescu, D., Stoican, F., Stamatescu, G., Ichim, L., Dragana, C., 2020. Advanced UAV–WSN system for intelligent monitoring in precision agriculture. Sensors 20 (3), https://doi.org/10.3390/s20030817.
Pournader, M., Shi, Y., Seuring, S., Koh, S.L., 2020. Blockchain applications in supply chains, transport and logistics: a systematic review of the literature. Int. J. Prod. Res. 58 (7), 2063–2081.
Primicerio, J., Di Gennaro, S.F., Fiorillo, E., Genesio, L., Lugato, E., Matese, A., Vaccari, F.P., 2012. A flexible unmanned aerial vehicle for precision agriculture.
Precis. Agric. 13 (4), 517–523. https://doi.org/10.1007/s11119-012-9257-6.
Pritchard, A., 1969. Statistical bibliography or bibliometrics. J. Document. 25 (4), 348–349.
Pudelko, R., Stuczynski, T., Borzecka-Walker, M., 2012. The suitability of an unmanned aerial vehicle (UAV) for the evaluation of experimental fields and crops. Agriculture 99 (4), 431–436.
Puri, V., Nayyar, A., Raja, L., 2017. Agriculture drones: a modern breakthrough in precision agriculture. J. Statis. Manag. Syst. 20 (4), 507–518.
Radoglou-Grammatikis, P., Sarigiannidis, P., Lagkas, T., Moscholios, I., 2020. A compilation of UAV applications for precision agriculture. Comput. Netw. 172,
107148 https://doi.org/10.1016/j.comnet.2020.107148.
Ramesh, K.V., Rakesh, V., Prakasa Rao, E.V.S., 2020. Application of big data analytics and artificial intelligence in agronomic research. Indian J. Agron. 65 (4), 383–395.
Raparelli, E., Bajocco, S., 2019. A bibliometric analysis on the use of unmanned aerial vehicles in agricultural and forestry studies. Int. J. Remote Sens. 40 (24),
9070–9083. https://doi.org/10.1080/01431161.2019.1569793.
Rasmussen, J., Nielsen, J., Garcia-Ruiz, F., Christensen, S., Streibig, J.C., Lotz, B., 2013.
Potential uses of small unmanned aircraft systems (UAS) in weed research. Weed Res. 53 (4), 242–248.
Rasmussen, J., Ntakos, G., Nielsen, J., Svensgaard, J., Poulsen, R.N., Christensen, S., Are vegetation indices derived from consumer-grade cameras mounted on
UAVs sufficiently reliable for assessing experimental plots? Eur. J. Agron. 74, 75–92. https://doi.org/10.1016/j.eja.2015.11.026.
Rejeb, A., Rejeb, K., Abdollahi, A., Zailani, S., Iranmanesh, M., Ghobakhloo, M., 2022. Digitalization in food supply chains: a bibliometric review and key-route main path
analysis. Sustainability 14 (1), 83. https://doi.org/10.3390/su14010083.
Rejeb, A., Rejeb, K., Simske, S.J., Treiblmaier, H., 2021a. Drones for supply chain management and logistics: a review and research agenda. Int. J. Logist. Res. Appl.
1–24. https://doi.org/10.1080/13675567.2021.1981273.
Rejeb, A., Rejeb, K., Simske, S., Treiblmaier, H., 2021b. Blockchain technologies in logistics and supply chain management: a bibliometric review. Logistics 5 (4), 72.
https://doi.org/10.3390/logistics5040072.
Rejeb, A., Rejeb, K., Simske, S., Treiblmaier, H., 2021c. Humanitarian drones: a review and research agenda. Internet of Things 16, 100434. https://doi.org/10.1016/j.
iot.2021.100434.
Rejeb, A., Treiblmaier, H., Rejeb, K., Zailani, S., 2021d. Blockchain research in healthcare: a bibliometric review and current research trends. J. of Data, Inf. and
Manag. 3 (2), 109–124.
Rejeb, A., Simske, S., Rejeb, K., Treiblmaier, H., Zailani, S., 2020. Internet of Things research in supply chain management and logistics: a bibliometric analysis. Internet
of Things 12, 100318.
ReportLinker, 2021. Global Agriculture Drones Market to Reach US$15.2 Billion by the YearGlobeNewswire News Room. https://www.globenewswire.com/news-release/2021/08/10/2277986/0/en/Global-Agriculture-Drones-Market-to-Reach-US-15-2-Billion-by-the-Year-2027.html.
Ribeiro-Gomes, K., Hernandez-L ´ opez, ´ D., Ortega, J.F., Ballesteros, R., Poblete, T.,Moreno, M.A., 2017. Uncooled thermal camera calibration and optimization of the
photogrammetry process for UAV applications in agriculture. Sensors (Switzerland) 17 (10). https://doi.org/10.3390/s17102173.
Rivera, M.A., Pizam, A., 2015. Advances in hospitality research: “From Rodney Dangerfield to Aretha Franklin”. Int. J. Contempor. Hospital. Manag. 27 (3),
362–378. https://doi.org/10.1108/IJCHM-03-2014-0146.
Roldan, ´ J.J., Joossen, G., Sanz, D., Del Cerro, J., Barrientos, A., 2015. Mini-UAV based sensory system for measuring environmental variables in greenhouses. Sensors 15 (2), 3334–3350. https://doi.org/10.3390/s150203334.
Rozenberg, G., Kent, R., Blank, L., 2021. Consumer-grade UAV utilized for detecting and analyzing late-season weed spatial distribution patterns in commercial onion fields. Precis. Agric. 22 (4), 1317–1332. https://doi.org/10.1007/s11119-021-09786-y.
Saari, H., Pellikka, I., Pesonen, L., Tuominen, S., Heikkila, ¨ J., Holmlund, C., Makynen, ¨ J., Ojala, K., Antila, T., 2011. Unmanned aerial vehicle (UAV) operated spectral camera system for forest and agriculture applications. Proceed. SPIE – Int. Soc. Opt. Eng. 8174 https://doi.org/10.1117/12.897585.
Sah, B., Gupta, R., Bani-Hani, D., 2021. Analysis of barriers to implement drone logistics. Int. J. Logist. Res. Appl. 24 (6), 531–550. https://doi.org/10.1080/
13675567.2020.1782862.
Saha, A. K., Saha, J., Ray, R., Sircar, S., Dutta, S., Chattopadhyay, S. P., & Saha, H. N., IOT-based drone for improvement of crop quality in agricultural field. In S. H.
N. Chakrabarti S. (Ed.), 2018 IEEE 8th Annual Computing and Communication Workshop and Conference, CCWC 2018 (Vols. 2018-January, pp. 612–615). Institute
of Electrical and Electronics Engineers Inc. doi: 10.1109/CCWC.2018.8301662.
Sai Vineeth, K.V., Vara Prasad, Y.R., Dubey, S.R., Venkataraman, H., 2019. LEDCOM: a novel and efficient LED based communication for precision agriculture. IEEE Conf. Info. Commun. Technol. 2019, 1–5. https://doi.org/10.1109/CICT48419.2019.9066177.
Salamí, E., Barrado, C., Pastor, E., 2014. UAV flight experiments applied to the remote sensing of vegetated areas. Remote Sensing 6 (11), 11051–11081. https://doi.org/10.3390/rs61111051.
Sankaran, S., Khot, L.R., Espinoza, C.Z., Jarolmasjed, S., Sathuvalli, V.R., Vandemark, G. J., Miklas, P.N., Carter, A.H., Pumphrey, M.O., Knowles, N.R.N., Pavek, M.J., 2015.
Low-altitude, high-resolution aerial imaging systems for row and field crop phenotyping: a review. Eur. J. Agron. 70, 112–123. https://doi.org/10.1016/j.
eja.2015.07.004.
Santesteban, L.G., Di Gennaro, S.F., Herrero-Langreo, A., Miranda, C., Royo, J.B., Matese, A., 2017. High-resolution UAV-based thermal imaging to estimate the
instantaneous and seasonal variability of plant water status within a vineyard. Agric. Water Manag. 183, 49–59. https://doi.org/10.1016/j.agwat.2016.08.026.
Sarli, C.C., Dubinsky, E.K., Holmes, K.L., 2010. Beyond citation analysis: A model for assessment of research impact. J. Med. Library Assoc. : JMLA 98 (1), 17–23. https://doi.org/10.3163/1536-5050.98.1.008.
Schaepman, M.E., Ustin, S.L., Plaza, A.J., Painter, T.H., Verrelst, J., Liang, S., 2009. Earth system science related imaging spectroscopy—an assessment. Remote Sens. Environ. 113, S123–S137.
Schirrmann, M., Giebel, A., Gleiniger, F., Pflanz, M., Lentschke, J., Dammer, K.-H., 2016. Monitoring agronomic parameters of winter wheat crops with low-cost UAV
imagery. Remote Sensing 8 (9). https://doi.org/10.3390/rs8090706.
Schmale III, D.G., Dingus, B.R., Reinholtz, C., 2008. Development and application of an autonomous unmanned aerial vehicle for precise aerobiological sampling above
agricultural fields. J. Field Rob. 25 (3), 133–147. https://doi.org/10.1002/rob.20232.
Shadrin, D., Menshchikov, A., Somov, A., Bornemann, G., Hauslage, J., Fedorov, M.,
Enabling precision agriculture through embedded sensing with artificial intelligence. IEEE Trans. Instrum. Meas. 69 (7), 4103–4113.
Shakhatreh, H., Sawalmeh, A.H., Al-Fuqaha, A., Dou, Z., Almaita, E., Khalil, I.,
Othman, N.S., Khreishah, A., Guizani, M., 2019. Unmanned Aerial Vehicles (UAVs): a survey on civil applications and key research challenges. IEEE Access 7,
48572–48634. https://doi.org/10.1109/ACCESS.2019.2909530.
Shakoor, N., Northrup, D., Murray, S., Mockler, T.C., 2019. Big data driven agriculture: big data analytics in plant breeding, genomics, and the use of remote sensing
technologies to advance crop productivity. Plant Phenome J. 2 (1), 1–8.
Sharma, B.K., Chandra, G., Mishra, V.P., 2019. Comparitive Analysis and Implication of UAV and AI in Forensic Investigations. In: Proceedings – 2019 Amity International
Conference on Artificial Intelligence. https://doi.org/10.1109/AICAI.2019.8701407.
Sharma, R., Shishodia, A., Gunasekaran, A., Min, H., Munim, Z.H., 2022. The role of artificial intelligence in supply chain management: mapping the territory. Int. J.
Prod. Res. 1–24. https://doi.org/10.1080/00207543.2022.2029611.
Shi, Y., Thomasson, J.A., Murray, S.C., Pugh, N.A., Rooney, W.L., Shafian, S., Rajan, N., Rouze, G., Morgan, C.L.S., Neely, H.L., Rana, A., Bagavathiannan, M.V.,
Henrickson, J., Bowden, E., Valasek, J., Olsenholler, J., Bishop, M.P., Sheridan, R., Putman, E.B., Popescu, S., Burks, T., Cope, D., Ibrahim, A., McCutchen, B.F.,
Baltensperger, D.D., Avant, R.V., Vidrine, M., Yang, C., Zhang, J., 2016. Unmanned aerial vehicles for high-throughput phenotyping and agronomic research. PLoS ONE
11 (7), e0159781.
Shuai, G., Martinez-Feria, R.A., Zhang, J., Li, S., Price, R., Basso, B., 2019. Capturing maize stand heterogeneity across yield-stability zones using Unmanned Aerial
Vehicles (UAV). Sensors 19 (20), 4446. https://doi.org/10.3390/s19204446.
Small, H., 1973. Co-citation in the scientific literature: a new measure of the relationship between two documents. J. Am. Soc. Info. Sci. 24 (4), 265–269.
Small, H., Rorvig, M.E., Lunin, L.F., 1999. Visualizing science by citation mapping. J. Am. Soc. Info. Sci. 50 (9), 799–813.
Soares, V.H.A., Ponti, M.A., Gonçalves, R.A., Campello, R.J.G.B., 2021. Cattle counting in the wild with geolocated aerial images in large pasture areas. Comput. Electron. Agric. 189, 106354 https://doi.org/10.1016/j.compag.2021.106354.
Srivastava, K., Pandey, P.C., Sharma, J.K., 2020. An approach for route optimization in applications of precision agriculture using UAVs. Drones 4 (3), 58. https://doi.org/ 10.3390/drones4030058.
Stafford, J.V., 2000. Implementing precision agriculture in the 21st century. J. Agric. Eng. Res. 76 (3), 267–275.
Su, J., Coombes, M., Liu, C., Guo, L., Chen, W.-H., 2018. Wheat drought assessment by remote sensing imagery using unmanned aerial vehicle. In 2018 37th Chinese Control Conference (CCC).
Su, J., Liu, C., Coombes, M., Hu, X., Wang, C., Xu, X., Li, Q., Guo, L., Chen, W.-H., 2018. Wheat yellow rust monitoring by learning from multispectral UAV aerial imagery.
Comput. Electron. Agric. 155, 157–166. https://doi.org/10.1016/j. compag.2018.10.017.
Su, Y., Wang, X., 2021. Innovation of agricultural economic management in the process of constructing smart agriculture by big data. Sustainable Comput. Inf. Syst. 31, 100579 https://doi.org/10.1016/j.suscom.2021.100579.
Sullivan, D.G., Fulton, J.P., Shaw, J.N., Bland, G.L., 2007. Evaluating the sensitivity of an unmanned thermal infrared aerial system to detect water stress in a cotton canopy. Trans. ASABE 50 (6), 1955–1962.
Sumesh, K.C., Ninsawat, S., Som-ard, J., 2021. Integration of RGB-based vegetation index, crop surface model and object-based image analysis approach for sugarcane yield estimation using unmanned aerial vehicle. Comput. Electron. Agric. 180, 105903 https://doi.org/10.1016/j.compag.2020.105903.
Suomalainen, J., Anders, N., Iqbal, S., Franke, J., Wenting, P., Bartholomeus, H., Becker, R., Kooistra, L., 2013. A light-weight hyperspectral mapping system for
unmanned aerial vehicles—the first results. In: 2013 5th Workshop on Hyperspectral Image and Signal Processing: Evolution in Remote Sensing (WHISPERS), pp. 1–4. https://doi.org/10.1109/WHISPERS.2013.8080721.
Suomalainen, J., Anders, N., Iqbal, S., Roerink, G., Franke, J., Wenting, P., Hünniger, D., Bartholomeus, H., Becker, R., Kooistra, L., 2014. A lightweight hyperspectral
mapping system and photogrammetric processing chain for unmanned aerial vehicles. Remote Sensing 6 (11), 11013–11030. https://doi.org/10.3390/
rs61111013.
Syeda, I.H., Alam, M.M., Illahi, U., Su’ud, M.M., 2021. Advance control strategies using image processing, UAV and AI in agriculture: A review. World J. Eng. 18 (4),
579–589. https://doi.org/10.1108/WJE-09-2020-0459.
Tahai, A., Rigsby, J.T., 1998. Information processing using citations to investigate journal influence in accounting. Inf. Process. Manage. 34 (2–3), 341–359.
Tang, Y., Dananjayan, S., Hou, C., Guo, Q., Luo, S., He, Y., 2021. A survey on the 5G network and its impact on agriculture: challenges and opportunities. Comput.
Electron. Agric. 180, 105895 https://doi.org/10.1016/j.compag.2020.105895.
Tantalaki, N., Souravlas, S., Roumeliotis, M., 2019. Data-driven decision making in precision agriculture: the rise of big data in agricultural systems. J. Agric. Food Info.
20 (4), 344–380.
Tao, H., Feng, H., Xu, L., Miao, M., Yang, G., Yang, X., Fan, L., 2020. Estimation of the yield and plant height of winter wheat using UAV-based hyperspectral images.
Sensors 20 (4), 1231.
Techy, L., Schmale III, D.G., Woolsey, C.A., 2010. Coordinated aerobiological sampling of a plant pathogen in the lower atmosphere using two autonomous unmanned aerial vehicles. J. Field Rob. 27 (3), 335–343. https://doi.org/10.1002/rob.20335.
Tetila, E.C., Machado, B.B., Astolfi, G., Belete, N.A.d.S., Amorim, W.P., Roel, A.R., Pistori, H., 2020. Detection and classification of soybean pests using deep learning
with UAV images. Comput. Electron. Agric. 179, 105836.
Thamm, H.-P., Menz, G., Becker, M., Kuria, D.N., Misana, S., Kohn, D., 2013. The Use of Uas for Assessing Agricultural Systems in AN Wetland in Tanzania in the—And WetSeason for Sustainable Agriculture and Providing Ground Truth for Terra-Sar X Data. In: ISPRS – International Archives of the Photogrammetry, Remote Sensing and Spatial Information Sciences, pp. 401–406. https://doi.org/10.5194/isprsarchivesXL-1-W2-401-2013.
Thelwall, M., 2008. Bibliometrics to webometrics. J. Info. Sci. 34 (4), 605–621.
Torres-Sanchez, ´ J., Lopez-Granados, ´ F., Pena, ˜ J.M., 2015. An automatic object-based method for optimal thresholding in UAV images: application for vegetation detection in herbaceous crops. Comput. Electron. Agric. 114, 43–52. https://doi.org/10.1016/j.compag.2015.03.019.
Torres-Sanchez, ´ J., Lopez-Granados, ´ F., Serrano, N., Arquero, O., Pena, ˜ J.M., Hassan, Q. K., 2015. High-throughput 3-D monitoring of agricultural-tree plantations with Unmanned Aerial Vehicle (UAV) technology. PLoS ONE 10 (6), e0130479.
Torres-Sanchez, ´ J., Pena, ˜ J.M., de Castro, A.I., Lopez-Granados, ´ F., 2014. Multi-temporal mapping of the vegetation fraction in early-season wheat fields using images from UAV. Comput. Electron. Agric. 103, 104–113. https://doi.org/10.1016/j. compag.2014.02.009.
Tsouros, D.C., Bibi, S., Sarigiannidis, P.G., 2019. A review on UAV-based applications for precision agriculture. Information (Switzerland) 10 (11). https://doi.org/10.3390/info10110349.
Tu, Y.-H., Phinn, S., Johansen, K., Robson, A., Wu, D., 2020. Optimising drone flight planning for measuring horticultural tree crop structure. ISPRS J. Photogramm.
Remote Sens. 160, 83–96. https://doi.org/10.1016/j.isprsjprs.2019.12.006
Tzounis, A., Katsoulas, N., Bartzanas, T., Kittas, C., 2017. Internet of Things in agriculture, recent advances and future challenges. Biosyst. Eng. 164, 31–48.
https://doi.org/10.1016/j.biosystemseng.2017.09.007.
Uddin, A., Singh, V.K., Pinto, D., Olmos, I., 2015. Scientometric mapping of computer science research in Mexico. Scientometrics 105 (1), 97–114.
UN., 2019. World population prospects 2019. https://population.un.org/wpp/ (Accessed on 15/04/2022).
Uto, K., Seki, H., Saito, G., Kosugi, Y., 2013. Characterization of rice paddies by a UAVmounted miniature hyperspectral sensor system. IEEE J. Sel. Top. Appl. Earth Obs.
Remote Sens. 6 (2), 851–860. https://doi.org/10.1109/JSTARS.2013.2250921. van der Merwe, D., Burchfield, D.R., Witt, T.D., Price, K.P., Sharda, A., 2020. Drones in
agriculture. Adv. Agron. 162, 1–30.
Velusamy, P., Rajendran, S., Mahendran, R.K., Naseer, S., Shafiq, M., Choi, J.-G., 2022.
Unmanned Aerial Vehicles (UAV) in precision agriculture: applications and challenges. Energies 15 (1), 217. https://doi.org/10.3390/en15010217.
Ventura, D., Bonifazi, A., Gravina, M.F., Belluscio, A., Ardizzone, G., 2018. Mapping and classification of ecologically sensitive marine habitats using Unmanned Aerial
Vehicle (UAV) imagery and Object-Based Image Analysis (OBIA). Remote Sensing 10 (9), 1331. https://doi.org/10.3390/rs10091331.
Verger, A., Vigneau, N., Ch´eron, C., Gilliot, J.-M., Comar, A., Baret, F., 2014. Green area index from an unmanned aerial system over wheat and rapeseed crops. Remote Sens. Environ. 152, 654–664. https://doi.org/10.1016/j.rse.2014.06.006.
Von Bueren, S.K., Burkart, A., Hueni, A., Rascher, U., Tuohy, M.P., Yule, I.J., 2015. Deploying four optical UAV-based sensors over grassland: challenges and
limitations. Biogeosciences 12 (1), 163–175. https://doi.org/10.5194/bg-12-163-2015.
Vuran, M.C., Salam, A., Wong, R., Irmak, S., 2018. Internet of underground things in precision agriculture: architecture and technology aspects. Ad Hoc Netw. 81,
160–173. https://doi.org/10.1016/j.adhoc.2018.07.017.
Wamba, S.F., Queiroz, M.M., 2021. Responsible artificial intelligence as a secret ingredient for digital health: bibliometric analysis, insights, and research directions.
Info. Syst. Front. 1–16.
Wang, L., Zhang, G., Wang, Z., Liu, J., Shang, J., Liang, L., 2019. Bibliometric analysis of remote sensing research trend in crop growth monitoring: A case study in China. Remote Sensing 11 (7). https://doi.org/10.3390/rs11070809.
White, H.D., Griffith, B.C., 1981. Author cocitation: A literature measure of intellectual structure. J. Am. Soc. Info. Sci. 32 (3), 163–171.
Xiang, H., Tian, L., 2011. Development of a low-cost agricultural remote sensing system based on an autonomous unmanned aerial vehicle (UAV). Biosyst. Eng. 108 (2), 174–190. https://doi.org/10.1016/j.biosystemseng.2010.11.010.
Xie, C., Yang, C., 2020. A review on plant high-throughput phenotyping traits using UAVbased sensors. Comput. Electron. Agric. 178, 105731 https://doi.org/10.1016/j.
compag.2020.105731.
Yao, H., Qin, R., Chen, X., 2019. Unmanned aerial vehicle for remote sensing applications—a review. Remote Sensing 11 (12). https://doi.org/10.3390/
rs11121443.
Yeom, S., 2021. Moving people tracking and false track removing with infrared thermal imaging by a multirotor. Drones 5 (3), 65. https://doi.org/10.3390/drones5030065.
Yue, J., Feng, H., Jin, X., Yuan, H., Li, Z., Zhou, C., Yang, G., Tian, Q., 2018. A comparison of crop parameters estimation using images from UAV-mounted
snapshot hyperspectral sensor and high-definition digital camera. Remote Sensing 10 (7), 1138. https://doi.org/10.3390/rs10071138.
Yue, J., Yang, G., Li, C., Li, Z., Wang, Y., Feng, H., Xu, B., 2017. Estimation of winter wheat above-ground biomass using unmanned aerial vehicle-based snapshot
hyperspectral sensor and crop height improved models. Remote Sensing 9 (7). https://doi.org/10.3390/rs9070708.
Zahawi, R.A., Dandois, J.P., Holl, K.D., Nadwodny, D., Reid, J.L., Ellis, E.C., 2015. Using lightweight unmanned aerial vehicles to monitor tropical forest recovery. Biol.
Conserv. 186, 287–295. https://doi.org/10.1016/j.biocon.2015.03.031. Zamora-Izquierdo, M.A., Santa, J., Martínez, J.A., Martínez, V., Skarmeta, A.F., 2019.
Smart farming IoT platform based on edge and cloud computing. Biosyst. Eng. 177,
4–17.
Zarco-Tejada, P.J., Diaz-Varela, R., Angileri, V., Loudjani, P., 2014. Tree height quantification using very high resolution imagery acquired from an unmanned aerial
vehicle (UAV) and automatic 3D photo-reconstruction methods. Eur. J. Agron. 55, 89–99. https://doi.org/10.1016/j.eja.2014.01.004.
Zhang, C., Craine, W.A., McGee, R.J., Vandemark, G.J., Davis, J.B., Brown, J., Hulbert, S. H., Sankaran, S., 2020. Image-based phenotyping of flowering intensity in coolseason crops. Sensors 20 (5), 1450. https://doi.org/10.3390/s20051450.
Zhang, C., Kovacs, J.M., 2012. The application of small unmanned aerial systems for precision agriculture: a review. Precis. Agric. 13 (6), 693–712. https://doi.org/
10.1007/s11119-012-9274-5.
Zhang, L., Zhang, H., Niu, Y., Han, W., 2019. Mapping maize water stress based on UAV multispectral remote sensing. Remote Sensing 11 (6), 605.
Zhang, X., Han, L., Dong, Y., Shi, Y., Huang, W., Han, L., Gonz´ alez-Moreno, P., Ma, H., Ye, H., Sobeih, T., 2019. A deep learning-based approach for automated yellow rust
disease detection from high-resolution hyperspectral UAV images. Remote Sensing 11 (13), 1554.
Zhao, X., Zhang, J., Huang, Y., Tian, Y., Yuan, L., 2022. Detection and discrimination of disease and insect stress of tea plants using hyperspectral imaging combined with wavelet analysis. Comput. Electron. Agric. 193, 106717 https://doi.org/10.1016/j. compag.2022.106717.
Zheng, A., Wang, M., Li, C., Tang, J., Luo, B., 2022. Entropy guided adversarial domain adaptation for aerial image semantic segmentation. IEEE Trans. G
Zheng, H., Cheng, T., Yao, X., Deng, X., Tian, Y., Cao, W., Zhu, Y., 2016. Detection of rice phenology through time series analysis of ground-based spectral index data. Field Crops Res. 198, 131–139. https://doi.org/10.1016/j.fcr.2016.08.027.
Zheng, J., Yang, W., 2018. Design of a precision agriculture leakage seeding system based on wireless sensors. Int. J. Online Eng. 14 (05), 184.
Zhou, L., Gu, X., Cheng, S., Yang, G., Shu, M., Sun, Q., 2020. Analysis of plant height changes of lodged maize using UAV-LiDAR data. Agriculture 10 (5), 146. https://
doi.org/10.3390/agriculture10050146.
Zhou, S., Chai, X., Yang, Z., Wang, H., Yang, C., Sun, T., 2021. Maize-IAS: A maize image analysis software using deep learning for high-throughput plant phenotyping. Plant Methods 17 (1), 48. https://doi.org/10.1186/s13007-021-00747-0.
Zhou, X., Zheng, H.B., Xu, X.Q., He, J.Y., Ge, X.K., Yao, X., Cheng, T., Zhu, Y., Cao, W.X., Tian, Y.C., 2017. Predicting grain yield in rice using multi-temporal vegetation
indices from UAV-based multispectral and digital imagery. ISPRS J. Photogramm. Remote Sens. 130, 246–255. https://doi.org/10.1016/j.isprsjprs.2017.05.003.
Zhou, Y., Xie, Y., Shao, L., 2016. Simulation of the core technology of a greenhousemonitoring system based on a wireless sensor network. Int. J. Online Eng. 12 (05),
43.
Zhou, Z., Majeed, Y., Diverres Naranjo, G., Gambacorta, E.M.T., 2021. Assessment for crop water stress with infrared thermal imagery in precision agriculture: a review
and future prospects for deep learning applications. Comput. Electron. Agric. 182, 106019 https://doi.org/10.1016/j.compag.2021.106019.